the Creative Commons Attribution 4.0 License.
the Creative Commons Attribution 4.0 License.
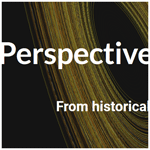
Review article: Interdisciplinary perspectives on climate sciences – highlighting past and current scientific achievements
Tommaso Alberti
Lesley De Cruz
Christian L. E. Franzke
Valerio Lembo
In the online seminar series “Perspectives on climate sciences: from historical developments to future frontiers”, which took place during 2020–2021, well-known and established scientists from several fields – including mathematics, physics, climate science and ecology – presented their perspectives on the evolution of climate science and on relevant scientific concepts. This special issue aims to create a platform for a more detailed elaboration of the topics discussed in the seminars but also to publish new scientific findings. In this paper, we first give an overview of the content of the seminar series, and then we introduce the written contributions to this special issue. In line with the spirit of the seminar series, this paper is structured along thematic areas of the broad field of climate science, conveying different perspectives on the climate system: geophysical fluid dynamics, dynamical systems theory, multiscale processes, statistical physics, paleoclimate and the human dimension.
- Article
(376 KB) - Full-text XML
- BibTeX
- EndNote
The “Perspectives on climate sciences: from historical developments to future frontiers” online seminar series (POCS, 2021) took place from September 2020 to July 2021, in the middle of the COVID-19 pandemic. The seminar series materialised from our belief that it is important for early-career scientists (ECSs) to learn more about their research field's broader scientific background and historical context. Positioning their own research in the “big picture” gives them additional motivation to pursue their scientific goals and shows them possible scenarios for the future evolution of climate sciences. Especially engaging for young researchers is to learn about the history of their research field directly from the pioneers who actively shaped the path of scientific progress. With this intention in mind, we invited well-known and well-established scientists to speak in front of a broad audience, including, but not restricted to, ECSs. They were asked to talk about their contribution to and perspective on the development of climate sciences from both a scientific and personal perspective, including the challenges they faced as young scientists. It was a great opportunity to gain insight into the scientific career paths of the speakers that were often far from straightforward. Their careers were hindered by many unforeseen difficulties, even self-doubt, but at the same time pushed forward by perseverance, dedication to science and sometimes a bit of luck. To many of us, especially ECSs, this gave more self-confidence and personal motivation, which were sorely needed in times of isolation due to the COVID-19 lockdowns and geopolitical turmoil.
Climate science emerges at the interface of several disciplines, such as physics, mathematics, chemistry, biology and the sphere of social sciences. Hence, it is highly interdisciplinary. A further aim of the seminar series was to stimulate the interaction among these disciplines. In David Ruelle’s seminar, we learned that, in his opinion, the most productive period in terms of interdisciplinary work took place between 1970 and 2000 and is connected to the development of chaos theory, building upon seminal works of mathematicians, physicists and meteorologists, like Henri Poincaré and Ed Lorenz. After this period, each discipline developed mainly along their own paths, drifting apart from each other. Nowadays, we are facing what is probably the biggest challenge of humankind: the ongoing global climate crisis. In order to be able to address this existential crisis, we need to exchange ideas and methods among the above disciplines and enhance interdisciplinary interactions. The successes achieved in the 1990s in closing the ozone hole in the Arctic stratosphere, as outlined in Susan Solomon's seminar, give us confidence that this is indeed possible and achievable.
The recognition of the well-posedness and rigorous mathematical background of the physics behind climate science was formalised in 2021, when the Nobel Prize in Physics was awarded to Klaus Hasselmann, Syukuro Manabe and Giorgio Parisi (Franzke et al., 2022). This was a milestone in the acknowledgement of the necessary relation between these disciplines, i.e. the broad cohort of science, technology, engineering and mathematics (STEM) on one side and human sciences on the other side. In his seminar, Hans von Storch reviewed the issue of post-normal science and basic norms for natural scientists (so-called CUDOS, referring to communalism, universalism, disinterestedness and organised scepticism), while Klaus Hasselmann advocated for the social role of the climate scientist in his interview. Eugenia Kalnay updated us on the newest developments in terms of coupling between Earth system models (ESMs) and human system models (HSMs). All together, these precious contributions emphasised the uncomfortable but challenging situation in which the climate scientist (meant in the broadest possible sense) is required to engage in the interaction with stakeholders and policymakers within and beyond the long-standing format of the Intergovernmental Panel for Climate Change (IPCC), jointly established by the World Meteorological Organization (WMO) and the United Nations Environment Programme (UNEP) in 1988.
The aim of this special issue is to create a platform for a more detailed elaboration of the topics discussed in the seminars but also to publish new scientific findings. In this paper, we first give an overview of the content of the seminar series in Sect. 2, and then we introduce the written contributions to this special issue in Sect. 3.
The interdisciplinary background of the speakers revealed several different perspectives on the climate system and climate science. In this section, we present a short overview of the content of the seminars in line with these perspectives. The geophysical fluid dynamics (GFD) perspective is a fundamental one. It provides the mathematical equations describing the evolution of the climate system and its main components and thus the basis for weather and climate modelling. The dynamical systems perspective focuses on the evolution of these equations – or rather of their reduced-order versions – in the phase space, using mathematical tools to analyse properties of different states of the system, such as stability and predictability. The multiscale perspective complements the dynamical systems view, interpreting the weather and climate as a high-dimensional complex system exhibiting scale invariance. When modelling the climate system, a stochastic behaviour emerges due to the sensitive dependence on the initial conditions and the practical impossibility of resolving the processes down to the scale of viscosity. Thus, the stochastic perspective is indispensable to accurate weather predictions and a proper interpretation of climate model output. The human dimension introduces additional uncertainty to projecting the future evolution of the system, highlighting at the same time that humans are an essential system component.
2.1 The climate system from the perspective of geophysical fluid dynamics
In order to understand the complex dynamics of the climate system beyond the radiation balance and to disentangle internal variability from external forcing, one needs to model the time evolution of the climate system's components and their interactions. These include the hydrosphere, the cryosphere, the lithosphere, the biosphere and – that which affects society most on a daily basis – the atmosphere. The GFD perspective describes the evolution of these components based on the principles of mechanics and thermodynamics. These principles can be expressed as partial differential equations in an appropriate form of the Navier–Stokes equations, adapted to the relevant processes at these vast scales, such as stratification and the Coriolis effect.
In order to describe the evolution of an immensely complex system such as the atmosphere and to gain insight into its dynamics, the relevant degrees of freedom and conserved quantities need to be identified. Brian Hoskins recounted how the concepts of (quasi-geostrophic) potential vorticity (Rossby, 1938, 1940) enabled the development of the first numerical weather prediction models and how isentropic potential vorticity maps helped gain an understanding of weather developments (Hoskins et al., 1985). A century before the introduction of these concepts, severe weather phenomena such as hurricanes had already motivated early meteorologists to analyse their rotating behaviour on weather maps and to understand their dynamics. Kerry Emanuel described how the scientific progress around hurricanes took a step backwards, highlighting a persistent misunderstanding around the processes driving these dynamics. Despite progress made in the 1940s and 1950s (Kleinschmidt, 1951; Riehl, 1950), later research ignored the importance of surface enthalpy fluxes and instead focused on latent heat release. This led to a failure of numerical simulations of hurricanes and the persistence of errors in textbook descriptions even until today. Finally, improved observations played a key role in confirming the dominant drivers.
Raymond Pierrehumbert pointed out in his talk that as simple as the rules governing an atmosphere with water vapour might be – i.e. Newtonian mechanics, thermodynamics and radiative transfer – nontrivial properties emerge in the resulting climate system. Again, misconceptions such as the thermostat hypothesis remind us to stay vigilant and encourage us to try reproduce earlier work. This hypothesis, which claimed that the temperature in the tropics could not exceed 302 K due to shading by high convective clouds, was debunked with some effort (Pierrehumbert, 1995). In his more recent work, Pierrehumbert (2005) showed how GFD ideas can be revisited on the new testing ground provided by the rich variety of exoplanetary atmospheres, including tidally locked planets and atmospheres with supercritical water.
On our own planet, again, Clara Deser explained how climate projections driven by GFD simulations help understand regional climate change effects. On these scales, internal variability has an enormous impact on the observed local trends in atmospheric variables. In her seminar, she presented a solution to robustly disentangle the effects due to the external forcing (anthropogenic, volcanic, etc.), the model differences and the system's internal stochastic variability: the latter can be identified as the variability observed in large initial-condition ensembles of historical climate simulations (Deser et al., 2012, 2020).
The disentangling of internal and forced variability was also the central theme of Michael Mann's story of the Atlantic Multidecadal Oscillation. This concept emerged based on the multidecadal (50–70 years) spectral peak in global surface temperature records and rapidly gained traction in scientific works but was subsequently shown to be an artefact caused by the response of the climate system to both anthropogenic and natural forcings rather than an internal mode of variability. Strong evidence for this was provided by the continued presence of this spectral peak in the globally averaged ensemble mean temperatures of CMIP5 (Coupled Model Intercomparison Project Phase 5, Taylor et al., 2012) climate control simulations of the last millennium. Indeed, one would expect the internal variability to be averaged over these ensembles, meaning that any remaining variability can only be attributed to external forcings (Mann et al., 2021).
2.2 The climate system from the perspective of dynamical systems theory
The atmosphere and the climate system as a whole, i.e. the coupled atmosphere, hydrosphere, biosphere, lithosphere and cryosphere, are chaotic dynamical systems, given the sensitive dependence of their evolution on initial conditions (Ghil and Lucarini, 2020). David Ruelle discussed in his seminar the interdisciplinary origins of chaos theory. We learned that, at the beginning of the 20th century, the mathematician Henri Poincaré (Poincaré, 1908) had already explicitly considered the fact that the weather is sensitive to initial conditions. He stated that meteorologists are not able to predict the exact location of a cyclone because of imperfect and insufficient initial conditions, which cause the weather to be perceived as something random. However, the chaotic nature of the weather did not become generally established until the work of Ed Lorenz (Lorenz, 1963) and the works that followed, all strongly connected to the spread of numerical computers in the middle of the 20th century. As David Ruelle reported, this was followed by an explosion of interdisciplinary work on chaos theory between 1970 and 2000 involving meteorology, hydrodynamics, chemical kinetics and the astronomy of the solar system (Ruelle and Takens, 1971; Li and Yorke, 1975; Laskar, 1989). After that, interdisciplinarity declined, and the work nowadays is mainly done in individual disciplines.
In contrast to the GFD perspective, which focuses on equations describing the temporal evolution of dynamic and thermodynamic variables, the dynamical system perspective considers the solution of these equations in the phase space, the set of all possible configurations of the system. In the phase space, one studies trajectories corresponding to different initial conditions, the stability of fixed points, periodic and non-periodic orbits, the geometry of the attractor, and the properties of the invariant measure. Despite the high level of mathematical abstraction, these concepts are useful because they provide unique insights into the system’s dynamics. It becomes clear, for example, that the geometrical properties of the attractor are strongly related to the evolution of trajectories and thus to the dynamics of the system. Similar states or configurations of the climate system are close to each other in the phase space and evolve correspondingly with the local geometry of the attractor in that region, even if they are far away from each other in time. However, as we found out in James Yorke’s seminar, the dynamics of a chaotic system can be very complex due to phenomena such as hetero-chaos (Saiki et al., 2018, 2021). Because of this, very similar initial conditions can lead to completely different evolutions of the weather and thus to serious predictability issues.
These predictability issues are well-known in numerical weather prediction, where it has become clear over time that purely deterministic predictions are insufficient and misleading. Tim Palmer convinced us in his seminar that the predictability of a nonlinear system and the related uncertainty always depend on the initial condition. Thus, further developments in probabilistic prediction, based on enough ensemble members, are crucially important for the future of weather prediction. Also important for the future is exploiting the possibilities offered by artificial intelligence, as well as using stochasticity for improved parameterisations and data assimilation and for making simulations computationally less expensive.
Phenomena such as climate change, tipping points and internal variability cannot be explained based on the classical concept of an attractor with an invariant measure. In his seminar, Michael Ghil talked about pullback attractors of non-autonomous dynamical systems, i.e. with time-dependent forcing (Crauel and Flandoli, 1994; Ghil et al., 2008; Chekroun et al., 2011; Drótos et al., 2015). Already, the presence of noise in the form of internal variability makes the notion of a pullback attractor necessary. In the case of constant external forcing and stochastic natural forcing (internal variability), the corresponding attractor is a time-invariant pullback attractor, referred to as a random attractor. The effect of changing radiative forcing turns the attractor into a time-dependent pullback attractor. Denisse Sciamarella showed us how the study of the attractor's topology, with the help of branched manifolds, helps us to understand chaos and the effect of noise. Due to noise-induced chaos, the attractor can be described by different homology groups at different time steps (Sciamarella and Mindlin, 2001; Charó et al., 2021).
2.3 The climate system from a multiscale perspective
The climate system is a complex system featuring a large number of degrees of freedom with components that interact in a nonlinear way (Franzke and O'Kane, 2017; Dijkstra, 2013). These concepts are strictly related to those relying on the description of turbulent flows, such as the existence of a hierarchy of exponents to fully characterise the nature of the system (i.e. the so-called multifractal view; Frisch, 1995), the observation of a global- vs. local-scale invariance (Kuzzay et al., 2017) and the role of extreme events as singularities (Alberti et al., 2023).
Shaun Lovejoy applied the above concepts to describe different dynamical regimes in the climate system: the weather, the macroweather and the climate (Lovejoy, 2019). Indeed, weather and climate models are based on thermodynamics and continuum mechanics and are successful because they retain only some relevant “macroscopic” variables. However, the role of the “details” (see Mandelbrot, 1977) cannot be ignored, requiring the development of a higher-level description of unknown aspects of the climate system. This led to several (high-level) macroweather and climate models based on energy balance and scale invariance, which profoundly affect our forecasting capabilities at monthly and seasonal scales, as well as improved multidecadal climate projections.
Bérengère Dubrulle went deeper into the details by focusing on a ubiquitous aspect of fluids: turbulence (Dubrulle, 2022). Turbulent flows are characterised by a self-similar energy spectrum, the signature of fluid movements at all scales. This organisation has been described for more than 70 years by the phenomenology of the Kolmogorov–Richardson cascade (Kolmogorov, 1941; Richardson, 2007): the energy injected on a large scale by the work of the force that moves the fluid (e.g. a turbine) is transferred to smaller and smaller scales with a constant dissipation rate, up to the Kolmogorov scale, where it is transformed into heat and dissipated by viscosity. Such cascade phenomenology is at the basis of most turbulent models. Dubrulle further discussed how progress in numerical simulations and laboratory experiments gradually changed such a simple vision (starting from Landau's objection in the 1950s), leading to a new picture where quasi-singularities living beyond the Kolmogorov scale play a central role (Dubrulle et al., 2022). This has important impacts on resolution requirements of numerical simulations and calls for new models of turbulence (Dubrulle and Gibbon, 2022).
Ken Golden focused instead on a key component of Earth's climate system: the polar sea ice. Indeed, sea ice exhibits complexity on length scales ranging from tenths of millimetres to tens of kilometres. A principal challenge in modelling sea ice and its role in climate is how to use information about the small-scale structure to find the effective or homogenised properties on larger scales relevant to coarse-grained climate models. In other words, and strictly connected to previous concepts introduced by Shaun Lovejoy and Bérengère Dubrulle, how can we predict macroscopic behaviour from microscopic laws? Similar questions arise in statistical mechanics, materials science, and many other areas of science and engineering (Golden et al., 2020b). In his talk, Golden gave an overview of recent results, inspired by composite materials and statistical physics theories, on modelling effective behaviour in the sea ice system over a broad range of scales (Golden et al., 2020a).
He also discussed how physical sea ice processes influence microbial communities in the ice and upper ocean and vice versa. This work is helping to advance how sea ice is represented in climate models and to improve projections of the fate of the Earth's sea ice packs and the ecosystems they support.
2.4 The climate system from the perspective of stochastic processes and statistical physics
When modelling the climate system, a stochastic behaviour emerges due to the sensitive dependence on the initial conditions and the practical impossibility of resolving the processes down to viscosity. This makes the stochastic perspective indispensable for useful weather predictions, allowing specifically for the estimation of their uncertainty and a proper interpretation of climate model outputs. At the same time, stochastic methods are useful for different aspects of weather and climate modelling, such as parameterisations (i.e. the representation of the impact of the processes not resolved by the numerical model as function of processes that are resolved), to model certain behaviours and processes in the climate system and even to reduce computational costs. Due to its focus on many-particle systems and the expertise in connecting micro- and macroscales, statistical physics offers inspiration and practical tools for understanding the climate system.
As a format break from the other seminars, the authors interviewed Klaus Hasselmann, who won the Nobel Prize in Physics in 2021 (Franzke et al., 2022; Bohémier, 2022) together with Syukuro Manabe and Giorgio Parisi. Klaus Hasselmann pioneered the development of stochastic climate models (Hasselmann, 1976) and reduced-order models (Hasselmann, 1988) in order to detect climate change signals (Hasselmann, 1993). During the discussion, Klaus Hasselmann stressed the importance of his background in physics for his scientific career and how he opened up new research fields and jumped to new endeavours when he got excited by new ideas without ever losing curiosity and a sense of fun while doing research. His work on stochastic climate models led to the development of stochastic climate theory and stochastic parameterisations, now in use in operational models.
Cécile Penland used Klaus Hasselmann's model reduction approach to develop linear inverse models (Penland, 1989). Linear inverse models are linear dynamical models driven by stochastic noise. In her presentation, she described her scientific path and how she started using stochastic methods. She emphasised the concept of probability as a physical, conserved quantity and pointed out the necessity of using this concept more often in climate science. However, she also stressed that stochastic methods have become more widespread nowadays.
Roberto Benzi introduced the concept of stochastic resonance to explain past climate variability together with Giorgio Parisi (Benzi et al., 1982). In his presentation, he outlined the many difficulties and misconceptions he faced when first introducing the concept in the fields of both climate dynamics and nonlinear dynamical systems. In the meantime, stochastic resonance has been used in a large number of physical systems.
In his seminar, Giovanni Jona-Lasinio helped us understand the state of non-equilibrium based on macroscopic fluctuations theory. He argued that non-equilibrium is characterised by a variety of phenomena; thus, searching for a unique theory, such as the one describing classical thermodynamics, is prone to fail, and one has to restrict themselves to subclasses of problems. He pointed out that one of the major difficulties is defining adequate thermodynamic functionals in states far from equilibrium, and he showed how large deviation rates are useful in this regard. Large deviation theory is currently applied in climate studies (Gálfi et al., 2021).
2.5 A paleoclimate perspective on the climate system
Paleoclimate broadens our perspective on the climate system due to the very long timescales considered. These long timescales are essential to understanding the dynamics of the whole system, including the effect of the slow components, such as the hydrosphere and the biosphere.
Pascale Barconnot emphasised in her talk that knowledge about past climate states brings us closer to understanding future changes and helps us evaluate climate models. Based on paleoclimate modelling, climate models can be tested under different conditions than what we experience today. One can test the effect of using different sets of parameters, applying different forcings or conducting simulations based on different experimental protocols. All this is important in order to be confident that models can indeed simulate the climate of the future. The paleoclimate perspective reveals that the various evolution periods in the history of the climate system do not just have a distinct mean state but are instead the result of very different system dynamics, with different internal variability and feedbacks.
Valérie Trouet pointed out, based on tree ring studies, the strong interconnection between different climate system components, discussing the remote effects of the atmospheric dynamics in the upper troposphere on the vegetation growth and wildfires at the surface. Furthermore, she emphasised the devastating effects of fire suppression on the frequency and extension of Californian wildfires. Disturbances are often part of the natural dynamics of the system, and altering their natural occurrence frequency can lead to the opposite of the intended outcome.
2.6 The human dimension of the climate system
On the one hand, humans are an essential part of the climate system. Due to the emission of greenhouse gases, land use and other human activities disturbing the natural balance, they directly affect the state of the system. However, at the same time, the state of the climate influences human activities and societies. On the other hand, the human dimension represents a huge challenge for modelling the future development of the climate system due to the difficulty – or even impossibility – of describing human behaviour and the evolution of societies based on mathematical equations.
Building upon Hasselmann's seminal ideas about climate response and attribution, Gabriele Hegerl gave a retrospective survey of how ideas such as optimal fingerprinting and the detection and attribution of climate change were brought up by her and her colleagues, mentioning Ben Santer and Klaus Hasselmann among others. The rationale for identifying causes of climate change was limited not only by the emergence of an anthropogenic signal but also by the issue of separating forcings and feedbacks in order to address the feasibility of an orthogonal approach to the attribution of changes in specific forcings, e.g. greenhouse gases or aerosols. Spatial patterns of the expected forced response (after Hasselmann) were, in this respect, compared to observations in order to isolate the role of internal variability. New perspectives are currently being drawn in the field by starting to apply the ideas of attribution to the investigation of the impacts of climate change.
In a thought-provoking talk, Hans von Storch stressed the evolution of the study of climate through the 20th century and the first part of the 21st century, starting from the concept of climate determinism, aimed at justifying the European colonisation of Africa and Asia, and moving towards a proper, rigorous, method-based science, relying on CUDOS principles, and towards the issues of post-normal sciences. Von Storch suggested that the scientific process has been challenged by the increasing demand for science-motivated policies. At the same time, policymakers have been progressively asked to take decisions aimed at addressing climate change adaptation and mitigation. In this context, the climate scientist has been required to take a political stance, and motivating public opinion has been, at times, given higher priority than producing additional scientific results. Von Storch then gave tips on how climate scientists may navigate these troubled waters and what are, in his opinion, the prospects ahead.
The contributions by Susan Solomon and Eugenia Kalnay went deeper into how much the interaction between policymakers and climate scientists is relevant in order to address the challenges posed by the changing climate. Susan Solomon gave an insightful retrospective on the path to the Montreal Agreement, which led to a worldwide ban on emissions responsible for widening the ozone hole over Antarctica. This is regarded to be a pioneering step in the virtuous interaction between policymakers and scientists, with scientific evidence motivating the choice of the community of nations. This step has paved the way to the establishment of the United-Nations-led IPCC, the recurrent redaction of the Assessment Reports and the organisation of the Conference of Parties (COP).
Eugenia Kalnay's contribution focused on stressing the importance and urgency of integrating the human system into Earth system modelling. Not only is this related to an innovative approach to the redaction of socio-economic development pathways serving as boundary conditions in Earth system model exercises, but the human system, according to Kalnay, also has to be viewed as a module and should be integrated into the Earth system model via coupling to the other components, such as the atmosphere, hydrosphere and cryosphere. The task is becoming more urgent as most of the world's population is increasingly reliant on fossil fuel combustion, urbanisation and the continuous increase in gross domestic product. Unlike the usual approach in the climate model communities, the Earth system–human system coupling has to be bidirectional and target the specific subdomains. According to Kalnay, the main challenge embedded in this viewpoint is addressing socio-economic policies, especially those that involve the modification of the current social inequalities.
The contributions to this special issue emerged from the motivations of either elaborating with more details or complementing the topics discussed during the seminars, which resulted in a collection of both research and review articles and one brief communication.
A central problem of climate projections, driven by geophysical fluid dynamics simulations, is how to disentangle the internal variability of the climate system from the forced response. The contribution by Clara Deser (Deser and Phillips, 2023) involves the theoretical reasoning behind the necessity of implementing large-ensemble model simulations in order to solve this problem. The focus is on three main aspects: producing large ensembles with different initial conditions, the role of observations, and the technique of dynamical adjustment to remove the effect of the general circulation from observed records and to obtain a more reliable estimate of the forced response. As a case study, the recent trends in European climate are investigated.
Kalnay et al. (2023) focus on the importance of considering all components in the climate system for numerical weather prediction and point to the advantages of strongly coupled data assimilation improved by machine learning using models of different complexity. In particular, they start from a simple Lorenz model by detecting slow and fast modes of motion. Variational and ensemble coupled data assimilation methods are compared in a coupled quasi-geostrophic model (De Cruz et al., 2016), and the ensemble Kalman filter is chosen for comparing weakly and strongly coupled data assimilation in general circulation models of intermediate complexity. Finally, neural networks are employed to improve the effectiveness of correlation cut-off techniques in addressing data assimilation with small-size ensembles.
Michael Ghil and Denisse Sciamarella (Ghil and Sciamarella, 2023) discuss the relationship between climate science and dynamical systems theory. The theoretical concepts and methods have influenced climate science since the 1960s. Recently, increased developments benefited from advancements in computing resources and observational capabilities. The text further explores the contributions of nonlinear dynamics and the theory of non-autonomous and random dynamical systems to understanding the interplay between natural variability and anthropogenic climate change, as well as the role of algebraic topology in shedding light on this relationship. The review concludes by addressing tipping points and transitions in climatic behaviour under time-dependent forcing.
Dorrington and Palmer (2023) also take the dynamical systems perspective and study the interaction between stochastic forcing and regime dynamics in a barotropic β-plane model. They discuss a rather counter-intuitive result showing that stochastic forcing can increase the low-frequency variability of the system and thus the persistence of certain regimes. This example motivates us to better understand the impact of stochastic physics on regimes in full-blown climate models.
Shaun Lovejoy (Lovejoy, 2023) interprets the atmosphere from a multiscale perspective and reviews the development of scaling notions in atmospheric sciences. Until the 1980s, scaling was limited to self-similar cases. However, recent developments in multifractals and generalised scale invariance allowed for characterising and modelling strongly intermittent scaling processes and anisotropic systems. These generalisations are crucial for atmospheric applications. Scaling is now considered to be a general symmetry principle that helps define dynamical regimes in weather, macroweather, macroclimate and megaclimate. Anisotropic scaling systems, such as atmospheric stratification, require new definitions of scale to understand structures' morphologies systematically. The text also addresses challenges in widely accepting the scaling paradigm and its implications for alternative scaling approaches in weather and climate models, including long-range forecasts and climate projections.
Golden et al. (2023) focus on a specific but very important problem of climate modelling: the right representation of the polar sea ice in climate models. Sea ice is a multiscale composite material, exhibiting complexity on length scales ranging from tenths of millimetres to tens of kilometres. They show how to model the role of sea ice in the climate system by connecting microscales and macroscales based on Stieltjes functions.
The contribution of Jona-Lasinio (2023) is related to the very important though challenging task of connecting micro- and macroscales as well but from the perspective of statistical physics. The author gives an overview of macroscopic fluctuation theory, discussing large deviation rates in non-equilibrium physical systems, the differences between equilibrium and non-equilibrium states, and the applicability of this theory to the climate system.
The human dimension takes a central role in the brief communication by Hans von Storch (von Storch, 2023). This contribution is aimed at giving a wide overview of the social implications of climate sciences through recent history, from climate determinism giving a scientific background to western colonisation to the ideas of post-normal science and basic scientific norms, the so-called CUDOS principles of communalism, universalism, disinterestedness and organised scepticism.
The online seminar series gave insight into the history of major methodological developments to better understand and simulate the complex climate system. It also highlighted the importance of fundamental mathematical and physical science for making progress in understanding the climate system, which was recently prominently illustrated by the award of the 2021 Nobel Prize in Physics to the climate scientists Syukuro Manabe and Klaus Hasselmann and the physicist Giorgio Parisi (Franzke et al., 2022). In particular, Klaus Hasselmann and Giorgio Parisi used fundamental mathematical methods to understand climate change. The speakers in the seminar series and their contributions to this special issue also demonstrate that, even today, further fundamental methodological mathematical and physical advances are needed to improve our understanding of the complex climate system. Dealing with the additional complexity, which arises from integrating human behaviour as an active part of the physical system, requires new groundbreaking tools and concepts. We hope that the seminar series and this special issue will motivate such further developments.
The presentation slides of the seminars are available at https://sites.google.com/view/perspectivesonclimate/materials (Lembo et al., 2024).
VMG coordinated the writing. LDC wrote Sect. 2.1, VMG Sect. 2.2 and 2.5, TA Sect. 2.3, CLEF Sect. 2.4, and VL Sect. 2.6. All authors contributed to writing the other parts of the manuscript and to reviewing and editing the manuscript. All authors contributed to the conceptualization and project administration of the seminar series and the resulting review paper. VL created and maintained the website of the seminar series.
At least one of the (co-)authors is a member of the editorial board of Nonlinear Processes in Geophysics. The peer-review process was guided by an independent editor, and the authors also have no other competing interests to declare.
Publisher’s note: Copernicus Publications remains neutral with regard to jurisdictional claims made in the text, published maps, institutional affiliations, or any other geographical representation in this paper. While Copernicus Publications makes every effort to include appropriate place names, the final responsibility lies with the authors.
This article is part of the special issue “Interdisciplinary perspectives on climate sciences – highlighting past and current scientific achievements”. It is not associated with a conference.
We would like to thank Valerio Lucarini for encouraging us in organising this seminar series and also for the many fruitful discussions on this topic. We are grateful to the speakers of the seminars for their inspiring talks and also to the authors of the publications for their valuable contributions to this special issue. We would also like to thank Olivier Talangrand for guiding us through the editing and publication process of this special issue. We are thankful to the University of Hamburg for providing access to Zoom webinars which made possible the organisation of the online seminars.
Christian L. E. Franzke is supported by the Institute for Basic Science (IBS), Republic of Korea, under grant no. IBS-R028-D1. Lesley De Cruz acknowledges support from the Belgian Science Policy Office (BELSPO) through the FED-tWIN programme (grant no. Prf-2020-017). Vera Melinda Galfi acknowledges the support of the Water and Climate Risk Department, Institute of Environmental Studies, Vrije Universiteit Amsterdam.
This paper was edited by Olivier Talagrand and reviewed by Valerio Lucarini and one anonymous referee.
Alberti, T., Daviaud, F., Donner, R. V., Dubrulle, B., Faranda, D., and Lucarini, V.: Chameleon attractors in turbulent flows, Chaos, Solitons & Fractals, 168, 113195, https://doi.org/10.1016/j.chaos.2023.113195, 2023. a
Benzi, R., Parisi, G., Sutera, A., and Vulpiani, A.: Stochastic resonance in climatic change, Tellus, 34, 10–16, 1982. a
Bohémier, K. A.: Analysis for Science Librarians of the 2021 Nobel Prize in Physics: Climate, Spin Glass, and Complex Systems, Sci. Tech. Libr., 41, 1–23, 2022. a
Charó, G. D., Chekroun, M. D., Sciamarella, D., and Ghil, M.: Noise-driven topological changes in chaotic dynamics, Chaos, 31 10, 103115, https://doi.org/10.1063/5.0059461, 2021. a
Chekroun, M. D., Simonnet, E., and Ghil, M.: Stochastic climate dynamics: Random attractors and time-dependent invariant measures, Physica D, 240, 1685–1700, 2011. a
Crauel, H. and Flandoli, F.: Attractors for random dynamical systems, Probab. Theory Rel., 100, 365–393, 1994. a
De Cruz, L., Demaeyer, J., and Vannitsem, S.: The Modular Arbitrary-Order Ocean-Atmosphere Model: MAOOAM v1.0, Geosci. Model Dev., 9, 2793–2808, https://doi.org/10.5194/gmd-9-2793-2016, 2016. a
Deser, C. and Phillips, A. S.: A range of outcomes: the combined effects of internal variability and anthropogenic forcing on regional climate trends over Europe, Nonlin. Processes Geophys., 30, 63–84, https://doi.org/10.5194/npg-30-63-2023, 2023. a
Deser, C., Phillips, A., Bourdette, V., and Teng, H.: Uncertainty in climate change projections: the role of internal variability, Clim. Dynam., 38, 527–546, 2012. a
Deser, C., Lehner, F., Rodgers, K. B., Ault, T., Delworth, T. L., DiNezio, P. N., Fiore, A., Frankignoul, C., Fyfe, J. C., Horton, D. E., Kay, J. E., Knutti, R., Lovenduski, N. S., Marotzke, J., McKinnon, K. A., Minobe, S., Randerson, J., Screen, J. A., Simpson, I. R., and Ting, M.: Insights from Earth system model initial-condition large ensembles and future prospects, Nat. Clim. Change, 10, 277–286, 2020. a
Dijkstra, H. A.: Nonlinear climate dynamics, Cambridge University Press, https://doi.org/10.1017/CBO9781139034135, 2013. a
Dorrington, J. and Palmer, T.: On the interaction of stochastic forcing and regime dynamics, Nonlin. Processes Geophys., 30, 49–62, https://doi.org/10.5194/npg-30-49-2023, 2023. a
Drótos, G., Bódai, T., and Tél, T.: Probabilistic Concepts in a Changing Climate: A Snapshot Attractor Picture, J. Climate, 28, 3275–3288, 2015. a
Dubrulle, B.: Multi-Fractality, Universality and Singularity in Turbulence, Fractal and Fractional, 6, 613, https://doi.org/10.3390/fractalfract6100613, 2022. a
Dubrulle, B. and Gibbon, J. D.: A correspondence between the multifractal model of turbulence and the Navier-Stokes equations, Philos.T. R. Soc.Lond., 380, 20210092, https://doi.org/10.1098/rsta.2021.0092, 2022. a
Dubrulle, B., Daviaud, F., Faranda, D., Marié, L., and Saint-Michel, B.: How many modes are needed to predict climate bifurcations? Lessons from an experiment, Nonlin. Processes Geophys., 29, 17–35, https://doi.org/10.5194/npg-29-17-2022, 2022. a
Franzke, C. L. and O'Kane, T. J.: Nonlinear and stochastic climate dynamics, Cambridge University Press, https://doi.org/10.1017/9781316339251, 2017. a
Franzke, C. L., Blender, R., O'Kane, T. J., and Lembo, V.: Stochastic Methods and Complexity Science in Climate Research and Modeling, Front. Phys., 521, 931596, https://doi.org/10.3389/fphy.2022.931596, 2022. a, b, c
Frisch, U.: Turbulence: the legacy of AN Kolmogorov, Cambridge university press, https://doi.org/10.1017/CBO9781139170666, 1995. a
Gálfi, V. M., Lucarini, V., Ragone, F., and Wouters, J.: Applications of large deviation theory in geophysical fluid dynamics and climate science, La Rivista del Nuovo Cimento, 44, 291–363, https://doi.org/10.1007/s40766-021-00020-z, 2021. a
Ghil, M. and Lucarini, V.: The physics of climate variability and climate change, Rev. Mod. Phys., 92, 035002, https://doi.org/10.1103/RevModPhys.92.035002, 2020. a
Ghil, M. and Sciamarella, D.: Review article: Dynamical systems, algebraic topology and the climate sciences, Nonlin. Processes Geophys., 30, 399–434, https://doi.org/10.5194/npg-30-399-2023, 2023. a
Ghil, M., Chekroun, M. D., and Simonnet, E.: Climate dynamics and fluid mechanics: Natural variability and related uncertainties, Phys. D, 237, 2111–2126, 2008. a
Golden, K. M., Bennetts, L. G., Cherkaev, E., Eisenman, I., Feltham, D., Horvat, C., Hunke, E., Jones, C., Perovich, D. K., Ponte-Castañeda, P., Strong, C., Sulsky, D., and Wells, A. J.: Modeling sea ice, Not. Am. Math. Soc., 67, 1535–1555, https://doi.org/10.1090/noti2171, 2020a. a
Golden, K. M., Ma, Y., Strong, C., and Sudakov, I.: From Magnets to Melt Ponds, SIAM News, 53, 5–7, 2020b. a
Golden, K. M., Murphy, N. B., Hallman, D., and Cherkaev, E.: Stieltjes functions and spectral analysis in the physics of sea ice, Nonlin. Processes Geophys., 30, 527–552, https://doi.org/10.5194/npg-30-527-2023, 2023. a
Hasselmann, K.: Stochastic climate models part I. Theory, Tellus, 28, 473–485, 1976. a
Hasselmann, K.: PIPs and POPs: The reduction of complex dynamical systems using principal interaction and oscillation patterns, J. Geophys. Res.-Atmos., 93, 11015–11021, 1988. a
Hasselmann, K.: Optimal fingerprints for the detection of time-dependent climate change, J. Climate, 6, 1957–1971, 1993. a
Hoskins, B. J., McIntyre, M. E., and Robertson, A. W.: On the use and significance of isentropic potential vorticity maps, Q. J. Roy. Meteor. Soc., 111, 877–946, 1985. a
Jona-Lasinio, G.: Review article: Large fluctuations in non-equilibrium physics, Nonlin. Processes Geophys., 30, 253–262, https://doi.org/10.5194/npg-30-253-2023, 2023. a
Kalnay, E., Sluka, T., Yoshida, T., Da, C., and Mote, S.: Review article: Towards strongly coupled ensemble data assimilation with additional improvements from machine learning, Nonlin. Processes Geophys., 30, 217–236, https://doi.org/10.5194/npg-30-217-2023, 2023. a
Kleinschmidt, E.: Grundlagen einer Theorie der tropischen Zyklonen, Archiv für Meteorologie, Geophysik und Bioklimatologie, Serie A, 4, 53–72, 1951. a
Kolmogorov, A. N.: The local structure of turbulence in incompressible viscous fluid for very large Reynolds numbers, Cr Acad. Sci. URSS, 30, 301–305, 1941. a
Kuzzay, D., Saw, E.-W., Martins, F. J., Faranda, D., Foucaut, J.-M., Daviaud, F., and Dubrulle, B.: New method for detecting singularities in experimental incompressible flows, Nonlinearity, 30, 2381, https://doi.org/10.1088/1361-6544/aa6aaf, 2017. a
Laskar, J.: A numerical experiment on the chaotic behaviour of the Solar System, Nature, 338, 237–238, https://doi.org/10.1038/338237a0, 1989. a
Lembo, V., Alberti, T., De Cruz, L., Franzke, C., and Galfi, V. M.: Perspectives on Climate Sciences, https://sites.google.com/view/perspectivesonclimate/materials (last access: 28 March 2024), 2024. a
Li, T.-Y. and Yorke, J. A.: Period Three Implies Chaos, Am. Math. Mon., 82, 985–992, https://doi.org/10.2307/2318254, 1975. a
Lorenz, E. N.: Deterministic Nonperiodic Flow, J. Atmos. Sci., 20, 130–141, https://doi.org/10.1175/1520-0469(1963)020<0130:DNF>2.0.CO;2, 1963. a
Lovejoy, S.: Weather, Macroweather, and the Climate: Our Random Yet Predictable Atmosphere, Oxford University Press, https://doi.org/10.1093/oso/9780190864217.001.0001, 2019. a
Lovejoy, S.: Review article: Scaling, dynamical regimes, and stratification. How long does weather last? How big is a cloud?, Nonlin. Processes Geophys., 30, 311–374, https://doi.org/10.5194/npg-30-311-2023, 2023. a
Mandelbrot, B.: Fractals, Freeman San Francisco, ISBN-13 978-0716704737, 1977. a
Mann, M. E., Steinman, B. A., Brouillette, D. J., and Miller, S. K.: Multidecadal climate oscillations during the past millennium driven by volcanic forcing, Science, 371, 1014–1019, 2021. a
Penland, C.: Random forcing and forecasting using principal oscillation pattern analysis, Mon. Weather Rev., 117, 2165–2185, 1989. a
Pierrehumbert, R. T.: Thermostats, radiator fins, and the local runaway greenhouse, J. Atmos. Sci., 52, 1784–1806, 1995. a
Pierrehumbert, R. T.: Science Fiction Atmospheres, B. Am. Meteor. Soc., 86, 696–699, 2005. a
POCS: Perspectives on Climate Sciences home page, https://sites.google.com/view/perspectivesonclimate/home-page (last access: 19 September 2023), 2021. a
Poincaré, H.: Science et méthode, E. Flammarion, 1908. a
Richardson, L. F.: Weather prediction by numerical process, 2nd Edition, University Press, https://doi.org/10.1017/CBO9780511618291, 2007. a
Riehl, H.: A model of hurricane formation, J. Appl. Phys., 21, 917–925, 1950. a
Rossby, C.-G.: On the mutual adjustment of pressure and velocity distributions in certain simple current systems, II, J. Mar. Res., 1, 239–263, 1938. a
Rossby, C.-G.: Planetary flow pattern in the atmosphere, Q. J. Roy. Meteor. Soc., 66, 68–87, 1940. a
Ruelle, D. and Takens, F.: On the nature of trubulence, Commun. Math. Phys., 20, 167–192, https://doi.org/10.1007/BF01646553, 1971. a
Saiki, Y., Sanjuán, M. A. F., and Yorke, J. A.: Low-dimensional paradigms for high-dimensional hetero-chaos, Chaos, 28, 103110, https://doi.org/10.1063/1.5045693, 2018. a
Saiki, Y., Takahasi, H., and Yorke, J. A.: Piecewise linear maps with heterogeneous chaos, Nonlinearity, 34, 5744, https://doi.org/10.1088/1361-6544/ac0d45, 2021. a
Sciamarella, D. and Mindlin, G. B.: Unveiling the topological structure of chaotic flows from data, Phys. Rev. E, 643, 036209, https://doi.org/10.1103/PhysRevE.64.036209, 2001. a
Taylor, K. E., Stouffer, R. J., and Meehl, G. A.: An Overview of CMIP5 and the Experiment Design, B. Am. Meteor. Soc., 93, 485–498, https://doi.org/10.1175/BAMS-D-11-00094.1, 2012. a
von Storch, H.: Brief communication: Climate science as a social process – history, climatic determinism, Mertonian norms and post-normality, Nonlin. Processes Geophys., 30, 31–36, https://doi.org/10.5194/npg-30-31-2023, 2023. a