the Creative Commons Attribution 4.0 License.
the Creative Commons Attribution 4.0 License.
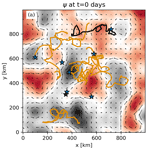
Inferring flow energy, space scales, and timescales: freely drifting vs. fixed-point observations
Aurelien Luigi Serge Ponte
Lachlan C. Astfalck
Matthew D. Rayson
Andrew P. Zulberti
Nicole L. Jones
A novel method for the inference of spatiotemporal decomposition of oceanic surface flow variability is presented and its performance assessed in a synthetic idealized configuration with horizontally divergentless flow. Inference methodology is designed for observations of surface velocity. The ability of networks of surface drifters and moorings to infer the spatiotemporal scales of surface ocean flow variability is quantified. The sensitivity of inference performance for both types of platforms to the number of observations, geometrical configurations, and flow regimes is presented. As drifters simultaneously sample spatial and temporal variability, they are shown to be able to capture both spatial and temporal flow properties even when deployed in isolation. Moorings are particularly adept for the characterization of the flow's temporal variability and may also capture spatial scales provided they are deployed as arrays. In particular, we show that our method correctly identifies whether drifters are preferentially sampling spatial vs. temporal variability. Pending further developments, this method opens novel avenues for the analysis of existing datasets as well as the design of future experimental campaigns targeting the characterization of small-scale (e.g., <100 km) ocean variability.
- Article
(2776 KB) - Full-text XML
-
Supplement
(14629 KB) - BibTeX
- EndNote
Characterizing oceanic surface motions in terms of their spatial and temporal scales is a recognized pathway toward the identification of the numerous processes that occur in the ocean as well as toward an improved understanding of their occurrences, life cycle, interactions, and impact on other components of the ocean variability (Ferrari and Wunsch, 2009). For example, Arbic et al. (2014) relied on horizontal wavenumber-frequency decompositions in order to quantify and rationalize the impact of ocean mesoscale turbulence on longer-term ocean variability in idealized, realistic numerical simulations and altimetric observations. At higher frequencies, wavenumber-frequency decomposition enables the separation of internal gravity waves and balanced motions that share similar spatial scales and are therefore entangled in instantaneous two-dimensional datasets (Torres et al., 2019; Jones et al., 2023). For example, using a wavenumber-frequency decomposition, Qiu et al. (2018) were able to quantify the so-called “transition scale” above which altimetric observations are dominated by balanced turbulence and below which smaller scales are dominated by internal gravity waves. These decompositions are easily performed with numerical simulation output and are provided on complete and regular spatial and temporal grids. However, the lack of observational knowledge of the high-frequency and small-scale distribution of energy is a recognized limitation of the validation of tide-resolving kilometer-resolution global- or basin-scale numerical models of the ocean circulation (Arbic et al., 2018; Yu et al., 2019b; Arbic et al., 2022).
The characterization of ocean variability in terms of spatial and temporal scales is also relevant from an operational perspective. The description of an ocean variable's autocorrelation properties is required to map sparse observations via optimal interpolation (Bretherton et al., 1976; Bretherton and McWilliams, 1980). For instance, estimation of surface currents heavily relies on the accurate mapping of altimetric observations which consist of narrow (order 5 to 10 km) geographically and temporally distant tracks (Pujol et al., 2016). The advent of wide-swath altimetric (Morrow et al., 2019; Fu et al., 2024) and upcoming current measuring satellite missions introduces novel challenges regarding the mapping of the observed variables and the separation of slower balanced motions and faster internal gravity waves. This has motivated the development of novel strategies for the separation of the signatures associated with both classes of motion. These strategies rely on a priori knowledge of the motions' spatial and temporal scales (Barth et al., 2014, 2021; Ubelmann et al., 2021, 2022).
The in situ characterization of ocean variability at mesoscale to submesoscale (e.g., <100 km, <10 d) has been a central objective for a number of ambitious experimental efforts over the last decade: LatMIX (Shcherbina et al., 2015), Carthe Consortium (Poje et al., 2014; D'Asaro et al., 2018), OSMOSIS (Buckingham et al., 2016; Yu et al., 2019a), and SMODE (Farrar et al., 2020). Estimation of the time–space decomposition of upper-ocean variability has resulted from the dense dedicated mooring deployments of OSMOSIS and further highlighted difficulties associated with the Doppler shifting of small-scale structures when observed from fixed platforms (Callies et al., 2020). Such experiments incur significant financial and environmental costs; therefore any optimization in the experimental design and/or improved data analysis strategies are advantageous. Drifters are cheap and experimentally light platforms for the spatial and temporal characterization of ocean variability but require adequate inference methodologies. This study presents one such methodological development.
The characterization of horizontal and temporal variability of oceanic surface motions from observations represents a challenge that depends on the class of motions of interest, the quantity and nature of observations available, and the lack of a methodology that is both sufficiently versatile to the differing observation platforms and mathematically coherent. Fixed-point platforms provide information that is horizontally localized over potentially extended time periods with fine temporal resolution. Such data are conducive to temporal decomposition (Polzin and Lvov, 2011). The tracking of surface and subsurface drifting platforms provides ocean current observations, which are also amenable to temporal decomposition (Lumpkin et al., 2017), albeit representing the Lagrangian particle and thereby aliasing certain spatial characteristics. At daily to monthly timescales, drifters have enabled characterization of mesoscale eddy variability via inspection of surface current autocorrelation or spectral properties (Zhang et al., 2001; Lumpkin et al., 2002; Veneziani et al., 2004; Sykulski et al., 2016) or rotary wavelet decomposition (Lilly and Gascard, 2006; Lilly et al., 2011). The Global Drifter Program has collected surface current information worldwide for ∼30 years. Recently, the advent of GPS and wider-bandwidth satellite communications has enabled high-frequency sampling of surface drifter positions and a generation of surface drifter velocity datasets with global hourly coverage (Elipot et al., 2016). Over the last decade, global descriptions of the ocean surface high-frequency variability have emerged (Elipot et al., 2010, 2016; Yu et al., 2019b; Arbic et al., 2022). These descriptions are timely to validate recent kilometer-scale tide-resolving basin-scale numerical simulations (Arbic et al., 2018).
Satellite observations are well posed to characterize surface ocean spatial variability. The constellation of conventional nadir altimeters provide maps of sea level and surface currents that resolve larger mesoscale motions (Ballarotta et al., 2019). However, spatial and temporal gaps between nadir altimeters impose limitations on the resolvable spatial and temporal resolutions (Ballarotta et al., 2023). Consequently, there are multiple spatial and temporal characterizations of ocean variability that combine altimetry with other in situ datasets, e.g., moorings, XBTs (expendable bathythermographs), and tomography (Zang and Wunsch, 2001; Wunsch, 2010; Wortham and Wunsch, 2014). For smaller spatial scales, ship-based measurements of tracers and currents have informed the estimation of spatial scales of ocean variability (Callies and Ferrari, 2013), but such measurements potentially entangle spatial and temporal contributions to an unclear extent. Drifters are thought to offer promising data for the description of smaller mesoscale and submesoscale variability (Balwada et al., 2016, 2021). Dedicated experiments with deployments of a large number of surface drifters such as the experiment conducted by the Carthe Consortium have provided useful datasets to demonstrate small-scale ocean variability despite also highlighting potential biases associated with the horizontally divergent character of the flow at these scales (Poje et al., 2017; Pearson et al., 2019, 2020; Wang and Bühler, 2021).
Here we present a new method for the spatial and temporal characterization of oceanic surface flow variability. To test the method we consider an idealized configuration of ocean variability, whose properties and synthetic generation are described in Sect. 2.1. The novel method for the inference of the flow properties is described in Sect. 2.3. The inference is then applied to several scenarios of observations in order to explore the performance of the inference relative to the number of observations (Sect. 3.2), to platform spatial separation (Sect. 3.1), and to flow regime (Sect. 3.4). The results are discussed and conclusions drawn in Sect. 4.
2.1 Idealized ocean surface flow design
We consider a two-dimensional and time-variable flow, described by the sum of rotational and divergent contributions:
where u and v are the zonal (toward positive x) and meridional (toward positive y) velocities in the respective directions x and y, ψ is the streamfunction, ϕ is the velocity potential, and ∂x and ∂y are the partial derivatives in x and y. We can describe the second-order behavior of ψ and ϕ, equivalently, by either their covariance functions or spectral densities. For general random fields a and b, defined over x, we define the stationary covariance function as , where the inner product is given as the covariance inner product . Here, the boldface x0 and τ denote a location and distance in x, respectively, in space and time. As stationarity is assumed, covariance is defined only as a function of τ. We define the corresponding spectral density as Sab(ω), where the boldface ω represents a location in wavenumber and frequency space. As shown by Wiener–Khinchin's theorem, the covariance function and the spectral density are Fourier pairs, so that
Given an assumed parameterization of Cψψ, Cϕϕ, and Cψϕ, the horizontal velocity auto- and cross-covariances are thus
Similarly, given the spectral densities Sψψ, Sϕϕ, and Sψϕ, we define the power and cross-power spectral densities of the horizontal velocities as
where k and l are horizontal wavenumbers. For our numerical experiment, we derive a purely rotational flow by setting ϕ=0 and, so, simply, and . This leads to the covariance functions , , and and spectral densities Suu=l2Sψψ, Svv=k2Sψψ, and .
To parameterize the flow we seek either a covariance function or spectral density that satisfies the physical requirements of the streamfunction ψ; namely, we require a log-linear decay in the high frequency or wavenumber of the spectral density. A good candidate for this is the isotropic Matérn covariance function (Rasmussen and Williams, 2005) with auto-covariance function and power spectral density (PSD),
where denotes the Euclidean norm/distance, D is the dimension of τ and ω, Γ(⋅) denotes the Gamma function, and 𝒦ν is the modified Bessel function of the second kind of order ν≥0. For positive half-integers of ν, i.e., , where , 𝒦ν has an analytical expression, otherwise it must be numerically calculated. We assume ψ to follow a separable Matérn process in space (D=2) and time (D=1), so that , where Ψ is the standard deviation of the streamfunction, , where τd represents the isotropic distance in space and τt represents the time lag, and both Css(τd) and Ctt(τt) are specified as correlation functions, that is, . For the kernel defined over space Css(τd), we define the slope and decorrelation parameters νs and λs, respectively. For the kernel defined over time Ctt(τt), we define the slope and decorrelation parameters νt and λt, respectively. This separability assumption is a concession on realism, which substantially eases the computational cost of the flow generation step and is not expected to affect our evaluation of the inference performance (Wortham and Wunsch, 2014; De Marez et al., 2023). The covariance functions with respect to u and v are thus
where primes denote derivatives with respect to the horizontal distance τd.
2.2 Synthetic flow data generation
The amplitude of the streamfunction Ψ is related to the flow standard deviation U via . The reference flow simulation is defined such as to be representative of moderately energetic mesoscale turbulence with U=0.1 m s−1, λs=100 km, and λt=5 d (Fig. 1) (Ferrari and Wunsch, 2009). Matérn slope parameters are chosen to be and , leading to a −2 temporal spectrum slope and a spatial isotropic spectral slope of −6. While the temporal spectral slope fits expectations, the spatial spectral slope is steeper by a value of 1 (20 %) compared to the value typical of quasi-geostrophic turbulence (Callies and Ferrari, 2013; Wortham and Wunsch, 2014). This concession to realism was made because it yields an analytical form for the Matérn covariance function, which alleviates the computational cost of the inference substantially. We reparameterize the covariance functions by Ψ=γλs, where γ is interpreted as the amplitude parameter of the horizontal velocity process; as well as interpretability, this has some computational benefits.
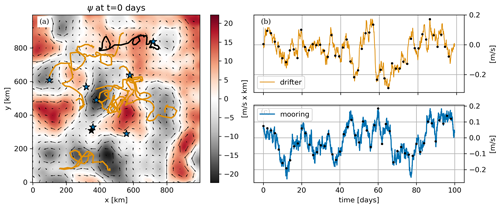
Figure 1Overview of the inference input data for the reference scenario: (a) streamfunction snapshot in color overlaid with eight drifter tracks and moorings used for the inference, (b) x velocity time series of the drifter identified by the black track in (a), and (c) x velocity time series at the mooring indicated by the black star in (a). In (b) and (c), black dots indicate the 2 d of sub-sampled data used in the inference.
With the previous choice of parameters, the streamfunction is generated over a 1000 km by 1000 km domain with 2 km grid spacing and over 100 d with hourly resolution (Fig. 1). This resolution is a factor of ∼50 times smaller than decorrelation, which is considered enough to resolve the synthesized variability and mitigate numerical interpolation errors in Lagrangian numerical simulations. Sizes of the spatial domain and the time series are ∼10 and ∼20 times larger than decorrelation scales, which ensures we are capturing multiple, effectively independent, realizations of the process.
The hourly synthetic flow is fed to the Parcels Python library configured with fourth-order Runge–Kutta time stepping and the default A-grid interpolation scheme in order to produce synthetic drifter trajectories (Delandmeter and van Sebille, 2019). Drifters are released initially at all flow grid points with the exception of a 20 km strip around all boundaries, amounting to a total of 9216 drifters for each simulation. Trajectories reaching domain boundaries are de-activated and not advected further in time and discarded from the list of observations that will be used for inference. The fraction of trajectories discarded was 52 % in the reference configuration. Drifter positions are stored at hourly resolution and velocities estimated from drifter positions with second-order finite differencing. An example of drifter trajectories is shown in Fig. 1. The flow amplitude averaged over time and space is about 1.8 % larger than that computed from drifter trajectories which reveals small turbophoresis, i.e., concentration of drifters in areas of lower energy (Freeland et al., 1975).
A non-dimensional parameter used to characterize flow is . This parameter is expected to control the relative importance of spatial vs. temporal variability in the projection onto Lagrangian time series (Middleton, 1985). In the reference scenario, the value of α is 0.4, which is in the range of observed ocean values (Lumpkin et al., 2002). In order to obtain mooring and drifter time series with different α values, the synthetic flow is simply rescaled, and new Lagrangian trajectories are simulated with the rescaled flow.
2.3 Inference
Observed data y are composed of flow time series collected over time by Np drifters or moorings to which a white noise n of standard deviation σ is added. The critical difference between drifter and mooring observations is that they are collected along drifter trajectories in the former case, i.e., u[x(t)]+n(t), where x(t) is a drifter trajectory, while they are collected at a fixed location in the latter one, i.e., where x is a mooring location.
We treat the collection of parameters as uncertain and unknown and probabilistically quantify this uncertainty. We treat Θ as a random variable and so naturally adopt the Bayesian paradigm of probability. Bayes' theorem states , where p(Θ∣y) is the posterior distribution, p(y∣Θ) is the likelihood, and p(Θ) is the prior distribution. The posterior is our target quantity and describes the probability distribution of Θ conditioned on the observed data. The likelihood is a probability distribution that assesses the probability of the data being generated, conditioned on some value of Θ. Finally, the prior represents our knowledge of Θ before we observe the data y; in this term we may include the results from previous analyses, bounds on values that Θ may take or any physically derived structure between the constituent parameters inside of Θ. Prior distributions are chosen here to be uniform between 0 and 10 times true parameter values.
Exact computation of p(Θ∣y) is analytically achievable for a small class of model problems; however, this is typically not so, and so p(Θ∣y) is computed numerically using Markov chain Monte Carlo (MCMC), as this is the gold standard in statistical computing. MCMC generates a dependent chain of draws from the posterior p(Θ∣y), such that subsets of Θ are visited proportionally to the posterior probability of the subsets. We show an example of this in Fig. 2 for the moored data reference scenario. The trace plots consist of 20 000 dependent samples from which we may derive summaries of the posterior distribution, p(Θ∣y), via standard Monte Carlo methods. For example, the marginal distributions of each parameter are represented by the histograms in the right-hand column of Fig. 2. MCMC is asymptotically exact in that the sampled draws converge to the exact posterior probability distribution. We generate samples using Metropolis–Hastings (MH), a well-known and accessible MCMC algorithm. Description and particulars are provided in the Appendix.
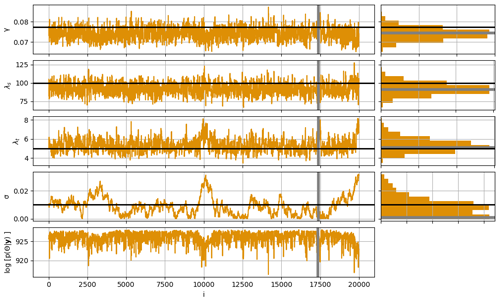
Figure 2Trace plots of MCMC sampling for the flow parameters (left) and associated histograms (right) for a single inference based on eight drifter trajectories for the reference scenario. True parameter values are indicated by the black lines, while MAP location and values are indicated by thick gray lines.
As discussed above, we parameterize our model using the Matérn covariance function as it exemplifies a number of desirable physical characteristics. However, the derivative of the Matérn covariance function is difficult to obtain due to 𝒦ν(⋅): analytical derivatives are only available at integer values of , and numerical calculations of 𝒦ν(⋅) are not available in any symbolic toolboxes that we are aware of. To mitigate the computational burden and enable the performance of ensemble of statistical experiments, we decided to fix the slope parameters νs and νt to half-integer values described in Sect. 2.2 and exclude these parameters from those inferred. This choice is relaxed in Sect. 3.5 in order to demonstrate that the inference of these parameters is possible as achieved in the purely temporal domain by Sykulski et al. (2016).
The noise chosen here (σ=0.01 m s−1) is representative of oceanographic velocity observations. State-of-the-art surface drifters are for instance equipped with GPS and provide hourly position observations with 10 m accuracy (Poulain et al., 2022). Under the assumption that the noise is white, this leads to a noise standard deviation on velocity observations of about 0.004 m s−1, which is less than half of what we considered. Acoustic Doppler current profilers (ADCPs), such as those deployed on moorings, exhibit typical accuracies in the 0.01 to 0.1 m s−1 range (Klema et al., 2020). Our expectation is that the performance of the inference should eventually decay as the noise on velocity observations increases, and this should be verified in a future study.
2.4 Validation of the inference methodology
As the mooring and drifter data are simulated, we know the ground truth and so may validate the MCMC sampling methodology. We show this for two cases: first, we show the probabilistic parameter estimates from the reference flow (Sect. 2.1), and second, we compare the maximum a posteriori (MAP) estimates, i.e., , of an 100-member ensemble with their true values. Examining a single scenario demonstrates the inherent uncertainty associated with a single experiment, whereas, inference across an ensemble looks at the variability that arises between data samples. In all cases, the data comprise a bivariate u and v time series collected either along eight trajectories (drifters) or at eight stationary locations (moorings), with 2 d temporal sampling over 100 d, amounting to 400 data points. The ensemble data are generated from the single spatiotemporal field with randomly sampled drifter tracks and mooring locations. Figure 2 shows the marginal posterior probability distributions of the single-member reference scenario. For all parameters, the true values lie well within the probability distribution. Note, σ2 is not well resolved; this is because of the roughness of the Matérn process at the set sampling interval (see Fig. 1), confounds with the noise signal so that both processes may be viable in explaining the observed data. This is somewhat expected, and more detailed statistical diagnostics accompany the code in the Supplement. Figure 3 plots a histogram of the MAP values calculated from each ensemble member's MCMC chain against the true value. This shows the variability of the distributions about the true value over the ensemble. Again, all distributions are centered on the true values, and there exists some difficulty in observing σ2 with precision. The precision of the inference will also be quantified by the difference between the third and first quartiles, which will be referred to as the interquartile width (IQW).
2.5 Inference scenarios
This study reports on the performance of the inference method under several scenarios (summarized in Table 1):
-
REF corresponds to the nominal configuration described in Sect. 2.4 with eight simultaneously deployed platforms.
-
Under SEP[dx], when multiple platforms are simultaneously sampling the flow, the separation between platforms and more generally their geometrical distribution is expected to modulate the performance of the inference. To simplify the analysis, we restrict the configuration to two simultaneous observing platforms (e.g., two drifters or two moorings) and investigate the sensitivity of the inference performance to their separation (with 10 % tolerance). For drifters, the separation is the initial one between the two drifters.
-
In the IND scenario, inference is performed by assuming time series from different platforms are independent from each other. Such a situation would occur if individual moorings/drifters were deployed at the same location, but at times sufficiently far apart, no correlation is expected across the velocity time series recorded by each platform. In effect this amounts to quantifying the ability of one platform at capturing flow parameters and investigating the sensitivity to the length of the time series.
-
Under OPT[Np], platforms are deployed in a spiral configuration that leads to separations that span the flow spatial decorrelation scale (see Sect. A2). The purpose of this experiment is to perform a simple experimental design optimization of the number of platforms deployed and of the choice between moorings and drifters.
-
In REG[α], the amplitude of the flow is rescaled in order to explore different values of the flow regime parameter . The amplitude of the noise is linearly scaled as a function of α in order to maintain a fixed signal-to-noise ratio. Inference is performed with a single platform.
-
The NU scenario is similar to OPT[Np] with Np=8, with the exception that the spectral slope parameters νs and νt are also inferred.
3.1 Platform separation sensitivity
Under scenario SEP[dx], estimations of the flow amplitude are comparable for observations from two moorings or two drifters and precise, with IQWs lower than 13 % of true amplitudes and no sensitivity to separation (Fig. 4a). We argue this follows from the fact that inferences are provided with velocity observations as inputs. Drifter inferences of the flow amplitude exhibit a 1 % to 3 % low bias, which is comparable to that associated with turbophoresis (Sect. 2.2).
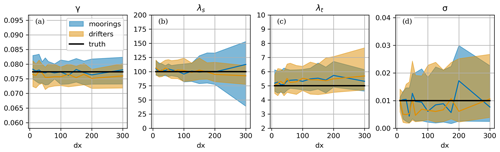
Figure 4Sensitivity of parameter MAP estimates to platform separation (in km) for the two platform configurations (scenario SEP[dx]). Lines represent the median, while shaded areas are bounded by first and third quartiles. True values are in black. When visible, gray shadings represent the no-go zone of the prior and inference parameter exploration.
Mooring spatial-scale estimates are sensitive to separation (Fig. 4b). After a modest decrease in performance of the inference with separation as measured by IQWs, the best inference is obtained for separation in the range of 40 to 80 km. For larger separations, the inference precision decreases, with IQW reaching values of about 0 % of true values at 300 km, i.e., 3 times the flow spatial scale. This loss of performance with separation reflects the loss of correlation between the flow measured by each mooring and thus the lack of information about spatial structure in the dataset. Drifters exhibit no clear sensitivity to separation for the spatial-scale estimate. This may first be explained by the substantial displacements of the drifters compared to the separations considered. A flow exponentially autocorrelated over 10 d with a standard deviation of 10 cm s−1 leads to an absolute dispersion of (250 km)2 (Gurarie et al., 2017). The natural ability of drifters to explore space and time and therefore constrain spatial scales (see Sect. 3.2) provides a second explanation.
Mooring and drifter inferences of the flow temporal scale both exhibit a modest high bias of 5 % to 10 % (Fig. 4c). As expected, drifters are overall less effective than moorings at estimating the flow temporal-scale parameter. IQWs associated with drifter inferences are systematically larger than those associated with moorings, which fluctuate around 30 % for moorings, compared with drifters, which increase with separation up to 60 %.
3.2 Sensitivity to the number of independent platforms
Under scenario IND[Np], single moorings (i.e., Np=1) provide estimates of the flow amplitude, γ, and temporal decorrelation scale, λt, parameters that are precise, with IQW starting at about 16 % and 45 % of true values, respectively. Parameters γ and λt converge to true values as the number of independent moorings is increased (Fig. 5). For the maximum number of platforms considered, the IQW of the flow amplitude and temporal decorrelation has decreased to 4 % and 11 %, respectively. As expected from their inability to explore the spatial dimension, single moorings are however globally unable to capture the flow spatial scale, with IQW comparable to half the width of the parameter space allowed to be explored, i.e., [0,1000 km], which amounts to the prior uncertainty (that is, there is no resolution of uncertainty).
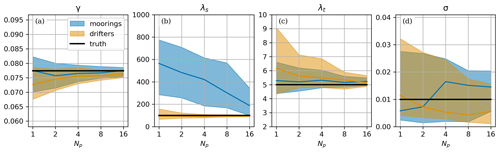
Figure 5Sensitivity of parameter MAP estimates to the number of platforms (scenario IND[Np]). Platforms are assumed independent from each other. Same representation as Fig. 4.
In comparison, drifters provide reasonable estimates of all three flow parameters (γ, λs, λt), with IQW starting at about 14 %, 92 %, and 95 % for one platform. These estimates converge toward truth as the number of platforms is increased, with IQW smaller than 16 % for all three parameters with 16 drifters. The ability of drifters to capture both spatial and temporal scales is explained by their ability to sample space and time simultaneously. MAP medians indicate mild biases with an underestimation of amplitude and overestimation of temporal decorrelation scale, which decrease as the number of drifters is increased. The amplitude low bias is about 7 % with a single drifter and reduces to about 1.4 % with 16 drifters, which is comparable to the turbophoresis bias (Sect. 2.2). The temporal decorrelation scale λt of drifters is always less accurate than that obtained with moorings, which we interpret as the price to pay for the simultaneous sampling of spatial and temporal variability.
3.3 Experimental design optimization
Optimizing an experimental design is a complex task that results from a compromise between scientific goals, a priori knowledge of the variables to be measured, financial and logistical constraints, and the need for redundancy, among other aspects. Scenario OPT[Np] illustrates how one could identify what the minimum experimental design is, enabling an accurate estimation of flow properties.
Consistent with the results of the previous scenarios, no substantial bias is observed. IQW is used to quantify accuracy and therefore is the target variable to minimize to identify optimal design (Fig. 6). Apart from the one platform configuration, where the mooring is unable to estimate the spatial scale of variability, moorings and drifters present comparable sensitivities as a function of the number of platforms. The number of platforms required to reach a target IQW of 20 % of the true value for all parameters except for σ is 4 for both platforms (Fig. 6).
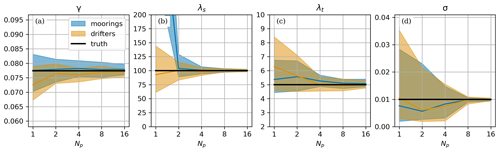
Figure 6Sensitivity of parameter MAP estimates to the number of platforms (scenario OPT[Np]). Same representation as Fig. 4.
In light of the low cost of drifters compared to moorings (factor of about 100 for deep sea applications), this result is particularly striking. However, we note the simplicity of the present exercise (idealized flow, constrained geometry of deployment; see Sect. A2) in light of past efforts on the matter (Bretherton and McWilliams, 1980; Barth and Wunsch, 1990). As stated in the preamble, optimizing for characterization of flow properties constitutes one consideration among many that may be taken in an experimental design optimization. Scientific goals may in general go well beyond the characterization of flow properties. If flow properties are suspected to evolve temporally, the use of drifters which are expected to eventually disperse will require multiple deployments in the area of interest unlike with moorings.
3.4 Flow regime sensitivity
We turn now to an investigation of the sensitivity of inferences to the flow parameter α (scenario REG[α]). We revert to the single-platform configuration in order to limit the exchange of information across platforms and the resulting constraint it brings for inference which may mask the α sensitivity. For comparison purposes we also perform a “time-only” inference of drifter velocity time series, which estimates flow amplitude, temporal decorrelation scale, and noise only and not the spatial decorrelation scale λs.
As anticipated from Sect. 3.2, inferences of flow amplitude and temporal decorrelation scale from mooring observations are relatively accurate, with IQW of about 15 % and 50 % of true values, respectively (Fig. 7a and c). The amplitude inference reflects the linear sensitivity to α. Spatial scales remain undetermined for all α values (Fig. 7b). This lack of sensitivity is expected due to exclusive sampling of temporal variability by a single mooring.

Figure 7Sensitivity of parameter MAP estimates to flow regime α for the single-platform configuration (scenario REG[α]). Time-only drifter inference is in red in (a) (median MAP dashed) and (c) (quartiles and median). Same representation as Fig. 4 otherwise.
Inferences of flow amplitude from drifter observations are comparable to mooring inferences in terms of IQW albeit with a low bias of about 5 % (Fig. 7a). A comparable bias is observed on time-only inferences for small α values but is exacerbated for α larger than unity where it reaches about 35 % of the true amplitude (Fig. 7c). For large α, distortions of the temporal spectrum shape are likely affecting the overall performance of the time-only inferences which rely on the spectral distribution following that of a Matérn process.
For small α values (<0.2), inference of the flow spatial decorrelation scale from drifter observations are the worst, and the IQW is nearly comparable to those from mooring observations (Fig. 7b). Drifters indeed merely move over a flow timescale comparable to the spatial decorrelation scale in this flow regime, which has been historically coined a “fixed float” and can be effectively considered a mooring (Middleton, 1985; Lumpkin et al., 2002). Accordingly, when α<0.2, estimates of the flow amplitude γ and temporal decorrelation scale λt are comparable for moorings and for drifters whether with the standard inference or the “time-only” inference.
For larger values of α (e.g., >0.2), the precision of the flow spatial decorrelation-scale inference from drifter observations improves substantially with decreasing IQW (down to 50 % at α∼1). In contrast, estimates of the temporal decorrelation scale deteriorate, with a bias high of about 25 % and IQW width of about 120 %. At these values of α, the flow is in the so-called “frozen turbulence” regime, and drifters are in effect experiencing the spatial variability of the flow field (Middleton, 1985; Lumpkin et al., 2002). This is directly reflected in the estimate of the temporal scale obtained from the “time-only” inference, which monotonically decreases with α. The fact that the temporal scale from the space–time inference does not follow a similar trend is a testimony to the relevance of the latter method, which is able to identify that observations reflect a predominance of spatial variability and attribute reasonable space-scale and timescale estimates, albeit with moderate error and bias.
3.5 Spectral slope estimation
For the final experiment (NU), the assumption that spectral slopes are known is relaxed, and Matérn slope parameters νs and νt are inferred along with the other parameters, i.e., γ, λs, λt, and σ. The assumed prior distributions are uniform over [1,5] and [0,5] for νs and νt, which is larger than typical uncertainties in the ocean for these parameters. Estimating these parameters leads to a 45-fold increase in computing time, due to computation of the Bessel function 𝒦ν, as discussed in Sect. 2.1.
The impact on flow parameter estimation is a modest increase of normalized IQW (Fig. 8) compared to OPT[Np] with Np=8 (Fig. 6). For instance, spatial and temporal decorrelation scales IQW estimated with mooring observations increase from 7 % and 14 % to 10 % and 18 % of true values, respectively. Inferences from drifter observations undergo comparable increases.
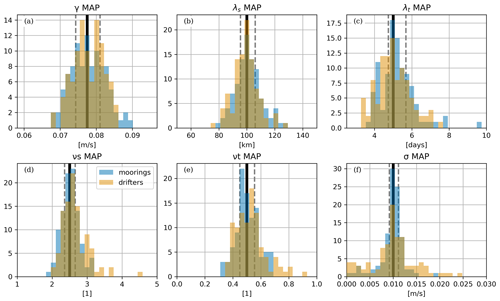
Figure 8Distribution of parameter MAP values for the reference flow and reference observation scenario with inference of Matérn spectral slopes (scenario NU). True parameter values are represented by vertical black lines. First and third quartiles of the posterior distribution are vertical dashed gray lines and provide insight into IQW.
The inference of spatial and temporal Matérn slopes is successful with posterior distributions centered around their true values and IQW of less than 22 % of true values. Independent experiments with random platform deployments similar to REF lead to more contrasted results, with the temporal slope being effectively resolved but not the spatial slope (not shown). This is an indication that the estimation of Matérn slope parameters is more demanding on observation quality and information content. Pending improvements in the performance of the inference computation, these results present promising perspectives for the systematic inference of spectral slopes.
We have presented a novel Bayesian method to infer surface ocean circulation spectral parameters (e.g., amplitude and spatial and temporal decorrelation scales) from sparse observations of the flow. The intention was to quantify parameter uncertainty due to sampling and flow regimes. These results may guide the design and analysis of future field campaigns and open novel avenues for the analysis of existing datasets. We considered flow observation from two platforms typically employed in oceanography: moorings which provide fixed-point flow observations and drifters that provide along-flow flow observations. Inference based on both types of platforms provides flow characterization estimates that converge to true values as the number of observations is increased. The performance of the method was quantified in various observing configurations, which allowed us to highlight the pros and cons of each type of platform. As already recognized, moorings are well suited to characterizing temporal scales of variability and if deployed as appropriately spaced simultaneous networks can constrain flow spatial scales. Drifters naturally sample both space and time, and we showed they can simultaneously constrain and separate the flow's space scales and timescales even when deployed in isolation, which is the first demonstration to our knowledge. We also showed that the ability of drifter observations to characterize flow properties depends on a non-dimensional parameter that quantifies the relative magnitude of the spatial and temporal decorrelation scales. Given the relative low cost and low environmental impact associated with drifter deployments compared with moorings, we argue they provide a powerful and more sustainable means to characterize surface flow properties. Finally, the present inference method may be of more general relevance for the simultaneous space–time characterization of flow properties in other fluid mechanics configurations (Reneuve and Chevillard, 2020).
More developments are required in order to make this method applicable to realistic oceanographic configurations. First the method needs to be extended to flows that are composed of a superposition of processes commonly occurring in the ocean, e.g., internal waves and tides, near-inertial waves. Such an extension will present methodological challenges associated with the parameterization of the space–time variability associated with these processes. The assumption of space–time separability, which was imposed here by the selected method of flow field generation, may have to be relaxed in a realistic configuration (Wortham and Wunsch, 2014; De Marez et al., 2023). As long as correlations may be expressed in physical space, extension of the inference to non-separable cases is direct. The issue introduced by non-separable kernels is arguably the most difficult challenge that needs to be addressed. It may also be useful to generalize the inference method to simultaneously account for observations that are of diverse nature, for instance current observations from drifters, pressure from moorings, and sea level observations from satellite altimetry. Such an extension will require deriving the expected correlation between each of the variables concerned and will in any case depend on the process modeled. A first application of the method to real data may be with gridded altimetric sea level or current data (AVISO+, 2024). The smoothing applied to generate these products may allow alleviation of the complexity associated with high-frequency processes.
Moving to a more realistic flow configuration will require evolving the synthetic flow strategy. The present choice allowed us to generate flows with arbitrary spatiotemporal structure, including some flows that are unlikely to occur in the ocean, in order to enable a broad exploration of the inference performance. This approach could be pushed further with the superposition of multiple processes and non-separable kernels and will likely require leveraging spectral domain approaches. As highlighted in Sect. 3.5, there are some computational difficulties with estimating the spectral slope via the Matérn covariance function. Slope estimation in the spectral domain is simple as the slope appears in the PSD in an analytically tractable form (see Sykulski et al., 2016); however, for drifter-based inference, as we are interested in estimation of the Eulerian properties, we cannot use such Lagrangian spectral techniques. There are some recent results that resolve the computational burden imparted by the calculation of the Bessel function and its derivatives (Geoga et al., 2022). Regrettably, at the time of writing, code for this study's methodology is not widely available across coding platforms. We hope that this, or similar methodological advancements, may be included in future work that will focus on estimating more realistic flows. Using flows generated from dynamical models (quasi-geostrophic, primitive equations) may eventually be necessary to capture regimes of variability more closely, representative of the actual ocean dynamics, with more realistic representations of process life cycles. Finally, the advection of drifters could account for a stochastic component in order to represent inaccuracies associated with the dynamical system considered (Mínguez et al., 2012).
Applications of the inference method to realistic observation datasets (e.g., velocity observations from the Global Drifter Program – Lumpkin et al., 2017) would be computationally prevented in the present form due to the use of dense covariance arrays. Alleviating this constraint will require us to leverage sparsity in the inference inputs associated with observations that are distant in space and/or time compared to associated decorrelation scales. This represents the second-most-important challenge that will have to be faced for applications in realistic configurations. Data collected from regional campaigns may be more suitable in the short term.
A1 MCMC sampling
A1.1 Metropolis–Hastings algorithm
The Markovian property of MCMC implies that a sample Θ[i] only depends on its previous sample Θ[i−1]; the method by which Θ[i] is generated from Θ[i−1] distinguishes the various MCMC algorithms. All MCMC algorithms propose some Θ[*] from Θ[i−1] and with probability α either accept Θ[*], in which case , or reject Θ[*], in which case . The Metropolis–Hastings (MH) algorithm, initially presented in Metropolis et al. (1953) and later extended by Hastings (1970), generates a proposal Θ[*] from Θ[i−1] using some user-specified proposal distribution . Given a proposal Θ[*], we accept the sample with probability r, where
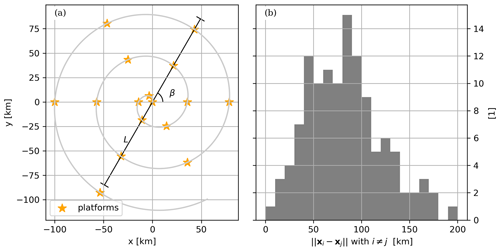
Figure A1(a) Illustration of an array of Np=16 platforms for L=200 km and used in OPT[Np]. (b) Corresponding distribution of platform separations.
If the proposal density is symmetrical, that is, , then Eq. (A1) reduces to the ratio of the posterior densities, and so the MH algorithm will always accept a proposed Θ[*] that is more probable than Θ[i−1]. The choice of is critical to the success of the MH algorithm. If is too wide, then the algorithm can become stuck for many iterations, thus generating very few unique proposals. Conversely, if is too narrow, the algorithm will not effectively explore the parameter space; the sampled will be highly correlated; and again, few independent samples will be generated. One of the main drawbacks of the MH algorithm is that there are sampling parameters that need to be hand-tuned.
We parameterize as a multivariate normal distribution with mean Θ[i−1] and diagonal covariance matrix. A widely agreed upon rule of thumb to balance exploration and exploitation of the posterior distribution is an acceptance probability of ∼0.25. Accordingly, we set the standard deviations of the proposal distribution to be between 0.05 and 0.2 of the true parameter values, corresponding to situations where we have larger and lower instances of observed data. The reason for this is simple: as the number of observations increases, the uncertainty of our parameter values decreases, implying a tighter posterior distribution. Consequently, a tighter proposal distribution is required to achieve a comparable acceptance probability. Full validation results to guarantee fit and convergence of the MCMC estimation algorithm are presented alongside the code at https://github.com/apatlpo/nwastats (last access: 31 July 2024).
A1.2 Notes on alternative MCMC sampling algorithms
Modern MCMC algorithms have been dominated by gradient-based proposal methods, where a proposal Θ[*] is generated by assessing the local topology surrounding Θ[i−1]: this allows the algorithm to efficiently trade off notions of exploration and exploitation of the posterior distribution. Included in these algorithms are the popular Hamiltonian Monte Carlo techniques, such as those implemented in Stan (Carpenter et al., 2017), PyMC3 (Salvatier et al., 2016), and Pyro (Bingham et al., 2019); these implementations, as well as others such as GPJax (Pinder and Dodd, 2022), will typically use symbolic toolboxes to define the local topology of the posterior. Alternative MCMC algorithms should not affect the accuracy of the posterior estimation; but rather, they will differ in their sampling efficiency. This study is concerned with inference, and not operationalization, and so we choose the Metropolis–Hastings algorithm so as to avoid the issue of gradients at the cost of some hand tuning of the algorithm.
A2 Platform array design
For the experiment OPT[Np], platforms are deployed at locations that aim to span a wide range of platform separations around some expectation of the spatial-scale decorrelation. For that purpose, locations were set along a spiral defined by its spatial footprint L, orientation β, and center (xc,yc) according to
where is a platform digit identifier. We have made the choice . An illustration of such platform deployment is illustrated in Fig. A1a. The distribution of platform separations successfully spans the ensemble of length scales up to L (Fig. A1b).
Each draw in the OPT[Np] ensemble experiment is based upon uniform random draws of the spiral center within the domain, of the spatial footprint L within [50 km,300 km], and of the orientation β within [0,2π].
Inferred parameters | |
γ | streamfunction amplitude to spatial- decorrelation-scale ratio |
λs | spatial decorrelation scale |
λt | temporal decorrelation scale |
σ | noise standard deviation |
νs | spatial slope parameter, only inferred in Sect. 3.5 |
νt | temporal slope parameter, only inferred in Sect. 3.5 |
Θ | vector composed of all inferred parameters |
Other parameters | |
U | flow amplitude |
Ψ | streamfunction amplitude |
non-dimensional flow parameter | |
Np | number of observing platforms (e.g., drifters or moorings) |
Variables | |
x,y | spatial coordinate or increment |
t | temporal coordinate or increment |
u,v | horizontal velocity field |
ψ | streamfunction |
ϕ | flow potential |
Cab | cross-correlation between variables a and b |
Sab | cross-spectrum between variables a and b |
(k,l) | horizontal wavenumbers |
𝒦ν | modified Bessel function of the second kind of order ν |
Γ | Gamma function |
y | observation vector |
The software code required to reproduce results is found at https://github.com/apatlpo/nwastats (last access: 31 July 2024) and https://doi.org/10.5281/zenodo.14261375 (Ponte, 2024).
All data in this work are synthetically generated. Details of the generation of the data are available in the referenced code, https://doi.org/10.5281/zenodo.14261375 (Ponte, 2024).
Animation of the synthetic flow and drifter trajectories in the REF, as well the REG[0.008] and REG[1.6] scenarios, is provided. The supplement related to this article is available online at: https://doi.org/10.5194/npg-31-571-2024-supplement.
All authors contributed to the conceptualization of this study. ALSP, LCA, MDR, and APZ developed the software required to perform the analysis. ALSP and LCA conducted the investigation. ALSP produced the visualization. ALSP and LCA prepared the original manuscript. ALSP, LCA, MDR, APZ, and NLJ reviewed and edited the manuscript. NLJ and ALSP acquired funding to make this work possible.
The contact author has declared that none of the authors has any competing interests.
Publisher's note: Copernicus Publications remains neutral with regard to jurisdictional claims made in the text, published maps, institutional affiliations, or any other geographical representation in this paper. While Copernicus Publications makes every effort to include appropriate place names, the final responsibility lies with the authors.
Aurelien Luigi Serge Ponte received support from the Institute of Advanced Studies (University of Western Australia) under the Gledden Visiting Fellowship program, as well as support from the TOSCA-ROSES SWOT project DIEGO. This research has been supported by the Australian Research Council (grant nos. IH200100009, LP210200613, and DP210102745).
This paper was edited by Jie Feng and reviewed by three anonymous referees.
Arbic, B. K., Müller, M., Richman, J. G., Shriver, J. F., Morten, A. J., Scott, R. B., Sérazin, G., and Penduff, T.: Geostrophic Turbulence in the Frequency–Wavenumber Domain: Eddy-Driven Low-Frequency Variability, J. Phys. Oceanogr., 44, 2050–2069, https://doi.org/10.1175/JPO-D-13-054.1, 2014. a
Arbic, B. K., Alford, M. H., Ansong, J. K., Buijsman, M. C., Ciotti, R. B., Farrar, J. T., Hallberg, R. W., Henze, C. E., Hill, C. N., Luecke, C. A., Menemenlis, D., Metzger, E. J., Müeller, M., Nelson, A. D., Nelson, B. C., Ngodock, H. E., Ponte, R. M., Richman, J. G., Savage, A. C., Scott, R. B., Shriver, J. F., Simmons, H. L., Souopgui, I., Timko, P. G., Wallcraft, A. J., Zamudio, L., and Zhao, Z.: A Primer on Global Internal Tide and Internal Gravity Wave Continuum Modeling in HYCOM and MITgcm, in: New Frontiers in Operational Oceanography, edited by: Chassignet, E. P., Pascual, A., Tintoré, J., and Verron, J., GODAE OceanView, https://doi.org/10.17125/gov2018.ch13, 2018. a, b
Arbic, B. K., Elipot, S., Brasch, J. M., Menemenlis, D., Ponte, A. L., Shriver, J. F., Yu, X., Zaron, E. D., Alford, M. H., Buijsman, M. C., Abernathey, R., Garcia, D., Guan, L., Martin, P. E., and Nelson, A. D.: Near-Surface Oceanic Kinetic Energy Distributions From Drifter Observations and Numerical Models, J. Geophys. Res.-Oceans, 127, e2022JC018551, https://doi.org/10.1029/2022JC018551, 2022. a, b
AVISO+: SSALTO/DUACS, https://www.aviso.altimetry.fr (last access: 26 September 2024), 2024. a
Ballarotta, M., Ubelmann, C., Pujol, M.-I., Taburet, G., Fournier, F., Legeais, J.-F., Faugère, Y., Delepoulle, A., Chelton, D., Dibarboure, G., and Picot, N.: On the resolutions of ocean altimetry maps, Ocean Sci., 15, 1091–1109, https://doi.org/10.5194/os-15-1091-2019, 2019. a
Ballarotta, M., Ubelmann, C., Veillard, P., Prandi, P., Etienne, H., Mulet, S., Faugère, Y., Dibarboure, G., Morrow, R., and Picot, N.: Improved global sea surface height and current maps from remote sensing and in situ observations, Earth Syst. Sci. Data, 15, 295–315, https://doi.org/10.5194/essd-15-295-2023, 2023. a
Balwada, D., LaCasce, J. H., and Speer, K. G.: Scale-dependent distribution of kinetic energy from surface drifters in the Gulf of Mexico, Geophys. Res. Lett., 43, 10856–10863, https://doi.org/10.1002/2016GL069405, 2016. a
Balwada, D., LaCasce, J. H., Speer, K. G., and Ferrari, R.: Relative Dispersion in the Antarctic Circumpolar Current, J. Phys. Oceanogr., 51, 553–574, https://doi.org/10.1175/JPO-D-19-0243.1, 2021. a
Barth, A., Beckers, J.-M., Troupin, C., Alvera-Azcárate, A., and Vandenbulcke, L.: divand-1.0: n-dimensional variational data analysis for ocean observations, Geosci. Model Dev., 7, 225–241, https://doi.org/10.5194/gmd-7-225-2014, 2014. a
Barth, A., Troupin, C., Reyes, E., Alvera-Azcárate, A., Beckers, J.-M., and Tintoré, J.: Variational interpolation of high-frequency radar surface currents using DIVAnd, Ocean Dynam., 71, 293–308, https://doi.org/10.1007/s10236-020-01432-x, 2021. a
Barth, N. and Wunsch, C.: Oceanographic Experiment Design by Simulated Annealing, J. Phys. Oceanogr., 20, 1249–1263, https://doi.org/10.1175/1520-0485(1990)020<1249:OEDBSA>2.0.CO;2, 1990. a
Bingham, E., Chen, J. P., Jankowiak, M., Obermeyer, F., Pradhan, N., Karaletsos, T., Singh, R., Szerlip, P., Horsfall, P., and Goodman, N. D.: Pyro: Deep Universal Probabilistic Programming, J. Mach. Learn. Res., 20, 1–6, 2019. a
Bretherton, F. P. and McWilliams, J. C.: Estimations from irregular arrays, Rev. Geophys., 18, 789–812, https://doi.org/10.1029/RG018i004p00789, 1980. a, b
Bretherton, F. P., Davis, R. E., and Fandry, C.: A technique for objective analysis and design of oceanographic experiments applied to MODE-73, Deep Sea Research and Oceanographic Abstracts, 23, 559–582, https://doi.org/10.1016/0011-7471(76)90001-2, 1976. a
Buckingham, C. E., Naveira Garabato, A. C., Thompson, A. F., Brannigan, L., Lazar, A., Marshall, D. P., George Nurser, A. J., Damerell, G., Heywood, K. J., and Belcher, S. E.: Seasonality of submesoscale flows in the ocean surface boundary layer, Geophys. Res. Lett., 43, 2118–2126, https://doi.org/10.1002/2016GL068009, 2016. a
Callies, J. and Ferrari, R.: Interpreting Energy and Tracer Spectra of Upper-Ocean Turbulence in the Submesoscale Range, J. Phys. Oceanogr., 43, 2456–2474, 2013. a, b
Callies, J., Barkan, R., and Naveira Garabato, A.: Time scales of submesoscale flow inferred from a mooring array, J. Phys. Oceanogr., 50, 1065–1086, https://doi.org/10.1175/JPO-D-19-0254.1, 2020. a
Carpenter, B., Gelman, A., Hoffman, M. D., Lee, D., Goodrich, B., Betancourt, M., Brubaker, M., Guo, J., Li, P., and Riddell, A.: Stan: A Probabilistic Programming Language, J. Stat. Softw., 76, 1–32, https://doi.org/10.18637/jss.v076.i01, 2017. a
D'Asaro, E. A., Shcherbina, A. Y., Klymak, J. M., Molemaker, J., Novelli, G., Guigand, C. M., Haza, A. C., Haus, B. K., Ryan, E. H., Jacobs, G. A., and Huntley, H. S.: Ocean convergence and the dispersion of flotsam, P. Natl. Acad. Sci. USA, 115, 1162–1167, 2018. a
De Marez, C., Callies, J., Haines, B., Rodriguez-Chavez, D., and Wang, J.: Observational Constraints on the Submesoscale Sea Surface Height Variance of Balanced Motion, J. Phys. Oceanogr., 53, 1221–1235, https://doi.org/10.1175/JPO-D-22-0188.1, 2023. a, b
Delandmeter, P. and van Sebille, E.: The Parcels v2.0 Lagrangian framework: new field interpolation schemes, Geosci. Model Dev., 12, 3571–3584, https://doi.org/10.5194/gmd-12-3571-2019, 2019. a
Elipot, S., Lumpkin, R., and Prieto, G.: Modification of inertial oscillations by the mesoscale eddy field, J. Geophys. Res.-Oceans, 115, https://doi.org/10.1029/2009JC005679, 2010. a
Elipot, S., Lumpkin, R., Perez, R. C., Lilly, J. M., Early, J. J., and Sykulski, A. M.: A global surface drifter data set at hourly resolution, J. Geophys. Res.-Oceans, 121, 2937–2966, https://doi.org/10.1002/2016JC011716, 2016. a, b
Farrar, J. T., D'Asaro, E., Rodriguez, E., Shcherbina, A., Czech, E., Matthias, P., Nicholas, S., Bingham, F., Mahedevan, A., Omand, M., Rainville, L., Lee, C., Chelton, D., Samelson, R., O'Neill, L., Lenain, L., Menemenlis, D., Perkovic-Martin, D., Mouroulis, P., Gierach, M., Thompson, D., Wineteer, A., Torres, H., Klein, P., Thompson, A., McWilliams, J. C., Molemaker, J., Barkan, R., Wenegrat, J., Rocha, C., Jacobs, G., D'Addezio, J., de Halleux, S., and Jenkins, R.: S-MODE: The Sub-Mesoscale Ocean Dynamics Experiment, in: IGARSS 2020–2020 IEEE International Geoscience and Remote Sensing Symposium, 26 September–2 October 2020, Waikoloa, Hawaii (online), 3533–3536, https://doi.org/10.1109/IGARSS39084.2020.9323112, 2020. a
Ferrari, R. and Wunsch, C.: Ocean Circulation Kinetic Energy: Reservoirs, Sources, and Sinks, Annu. Rev. Fluid Mech., 41, 253–282, 2009. a, b
Freeland, H., Rhines, P., and Rossby, T.: Statistical observations of the trajectories of neutrally buoyant floats in the North Atlantic, J. Mar. Res., 33, 383–404, 1975. a
Fu, L., Pavelsky, T., Cretaux, J., Morrow, R., Farrar, J. T., Vaze, P., Sengenes, P., Vinogradova-Shiffer, N., Sylvestre-Baron, A., Picot, N., and Dibarboure, G.: The Surface Water and Ocean Topography Mission: A Breakthrough in Radar Remote Sensing of the Ocean and Land Surface Water, Geophys. Res. Lett., 51, e2023GL107652, https://doi.org/10.1029/2023GL107652, 2024. a
Geoga, C. J., Marin, O., Schanen, M., and Stein, M. L.: Fitting Matérn Smoothness Parameters Using Automatic Differentiation, arXiv [preprint], https://doi.org/10.48550/arXiv.2201.00090, 2022. a
Gurarie, E., Fleming, C. H., Fagan, W. F., Laidre, K. L., Hernández-Pliego, J., and Ovaskainen, O.: Correlated velocity models as a fundamental unit of animal movement: synthesis and applications, Movement Ecology, 5, 13, https://doi.org/10.1186/s40462-017-0103-3, 2017. a
Hastings, W. K.: Monte Carlo sampling methods using Markov chains and their applications, Biometrika, 57, 97–109, https://doi.org/10.1093/biomet/57.1.97, 1970. a
Jones, C. S., Xiao, Q., Abernathey, R. P., and Smith, K. S.: Using Lagrangian Filtering to Remove Waves From the Ocean Surface Velocity Field, J. Adv. Model. Earth Sy., 15, e2022MS003220, https://doi.org/10.1029/2022MS003220, 2023. a
Klema, M. R., Pirzado, A. G., Venayagamoorthy, S. K., and Gates, T. K.: Analysis of acoustic Doppler current profiler mean velocity measurements in shallow flows, Flow Meas. Instrum., 74, 101755, https://doi.org/10.1016/j.flowmeasinst.2020.101755, 2020. a
Lilly, J. M. and Gascard, J.-C.: Wavelet ridge diagnosis of time-varying elliptical signals with application to an oceanic eddy, Nonlin. Processes Geophys., 13, 467–483, https://doi.org/10.5194/npg-13-467-2006, 2006. a
Lilly, J. M., Scott, R. K., and Olhede, S. C.: Extracting waves and vortices from Lagrangian trajectories, Geophys. Res. Lett., 38, L23605, https://doi.org/10.1029/2011GL049727, 2011. a
Lumpkin, R., Treguier, A.-M., and Speer, K.: Lagrangian Eddy Scales in the Northern Atlantic Ocean, J. Phys. Oceanogr., 32, 2425–2440, 2002. a, b, c, d
Lumpkin, R., Özgökmen, T., and Centurioni, L.: Advances in the application of surface drifters, Annu. Rev. Mar. Sci., 9, 59–81, 2017. a, b
Metropolis, N., Rosenbluth, A. W., Rosenbluth, M. N., Teller, A. H., and Teller, E.: Equation of State Calculations by Fast Computing Machines, J. Chem. Phys., 21, 1087–1092, https://doi.org/10.1063/1.1699114, 1953. a
Middleton, J. F.: Drifter spectra and diffusivities, J. Mar. Res., 43, 37–55, 1985. a, b, c
Mínguez, R., Abascal, A. J., Castanedo, S., and Medina, R.: Stochastic Lagrangian trajectory model for drifting objects in the ocean, Stoch. Env. Res. Risk A., 26, 1081–1093, https://doi.org/10.1007/s00477-011-0548-7, 2012. a
Morrow, R., Fu, L.-L., Ardhuin, F., Benkiran, M., Chapron, B., Cosme, E., d'Ovidio, F., Farrar, J. T., Gille, S. T., Lapeyre, G., Le Traon, P.-Y., Pascual, A., Ponte, A., Qiu, B., Rascle, N., Ubelmann, C., Wang, J., and Zaron, E. D.: Global Observations of Fine-Scale Ocean Surface Topography With the Surface Water and Ocean Topography (SWOT) Mission, Frontiers in Marine Science, 6, 232, https://doi.org/10.3389/fmars.2019.00232, 2019. a
Pearson, J., Fox-Kemper, B., Barkan, R., Choi, J., Bracco, A., and McWilliams, J. C.: Impacts of Convergence on Structure Functions from Surface Drifters in the Gulf of Mexico, J. Phys. Oceanogr., 49, 675–690, https://doi.org/10.1175/JPO-D-18-0029.1, 2019. a
Pearson, J., Fox-Kemper, B., Pearson, B., Chang, H., Haus, B. K., Horstmann, J., Huntley, H. S., Kirwan, A. D., Lund, B., and Poje, A.: Biases in Structure Functions from Observations of Submesoscale Flows, J. Geophys. Res.-Oceans, 125, e2019JC015769, https://doi.org/10.1029/2019JC015769, 2020. a
Pinder, T. and Dodd, D.: GPJax: A Gaussian Process Framework in JAX, Journal of Open Source Software, 7, 4455, https://doi.org/10.21105/joss.04455, 2022. a
Poje, A. C., Ozgokmen, T. M., Lipphardt, B. L., Haus, B. K., Ryan, E. H., Haza, A. C., Jacobs, G. A., Reniers, A. J. H. M., Olascoaga, M. J., Novelli, G., Griffa, A., Beron-Vera, F. J., Chen, S. S., Coelho, E., Hogan, P. J., Kirwan, A. D., Huntley, H. S., and Mariano, A. J.: Submesoscale dispersion in the vicinity of the Deepwater Horizon spill, P. Natl. Acad. Sci. USA, 111, 12693–12698, https://doi.org/10.1073/pnas.1402452111, 2014. a
Poje, A. C., Özgökmen, T. M., Bogucki, D. J., and Kirwan Jr., A. D.: Evidence of a forward energy cascade and Kolmogorov self-similarity in submesoscale ocean surface drifter observations, Phys. Fluids, 29, 020701, https://doi.org/10.1063/1.4974331, 2017. a
Polzin, K. L. and Lvov, Y. V.: Toward regional characterizations of the oceanic internal wavefield, Rev. Geophys., 49, RG4003, https://doi.org/10.1029/2010RG000329, 2011. a
Ponte, A.: apatlpo/nwastats: Publication Ponte et al. 2024 NPG (v0.1.0), Zenodo [code and data set], https://doi.org/10.5281/zenodo.14261375, 2024. a, b
Poulain, P.-M., Centurioni, L., and Özgökmen, T.: Comparing the Currents Measured by CARTHE, CODE and SVP Drifters as a Function of Wind and Wave Conditions in the Southwestern Mediterranean Sea, Sensors, 22, 353, https://doi.org/10.3390/s22010353, 2022. a
Pujol, M.-I., Faugère, Y., Taburet, G., Dupuy, S., Pelloquin, C., Ablain, M., and Picot, N.: DUACS DT2014: the new multi-mission altimeter data set reprocessed over 20 years, Ocean Sci., 12, 1067–1090, https://doi.org/10.5194/os-12-1067-2016, 2016. a
Qiu, B., Chen, S., Klein, P., Wang, J., Torres, H., Fu, L.-L., and Menemenlis, D.: Seasonality in Transition Scale from Balanced to Unbalanced Motions in the World Ocean, J. Phys. Oceanogr., 48, 591–605, https://doi.org/10.1175/JPO-D-17-0169.1, 2018. a
Rasmussen, C. E. and Williams, C. K. I.: Gaussian Processes for Machine Learning, The MIT Press, https://doi.org/10.7551/mitpress/3206.001.0001, 2005. a
Reneuve, J. and Chevillard, L.: Flow of Spatiotemporal Turbulentlike Random Fields, Phys. Rev. Lett., 125, 014502, https://doi.org/10.1103/PhysRevLett.125.014502, 2020. a
Salvatier, J., Wiecki, T. V., and Fonnesbeck, C.: Probabilistic programming in Python using PyMC3, PeerJ Computer Science, 2, e55, https://doi.org/10.7717/peerj-cs.55, 2016. a
Shcherbina, A. Y., Sundermeyer, M. A., Kunze, E., D'Asaro, E., Badin, G., Birch, D., Brunner-Suzuki, A. M. E., Callies, J., Cervantes, B. T. K., Claret, M., and Concannon, B.: The LatMix summer campaign: Submesoscale stirring in the upper ocean, B. Am. Meteorol. Soc., 96, 1257–1279, 2015. a
Sykulski, A. M., Olhede, S. C., Lilly, J. M., and Danioux, E.: Lagrangian Time Series Models for Ocean Surface Drifter Trajectories, J. Roy. Stat. Soc. C-App., 65, 29–50, https://doi.org/10.1111/rssc.12112, 2016. a, b, c
Torres, H., Klein, P., Siegelman, L., Qiu, B., Chen, S., Ubelmann, C., Wang, J., Menemenlis, D., and Fu, L.-L.: Diagnosing ocean-wave-turbulence interactions from space, Geophys. Res. Lett., 46, 8933–8942, 2019. a
Ubelmann, C., Dibarboure, G., Gaultier, L., Ponte, A., Ardhuin, F., Ballarotta, M., and Faugère, Y.: Reconstructing Ocean Surface Current Combining Altimetry and Future Spaceborne Doppler Data, J. Geophys. Res.-Oceans, 126, e2020JC016560, https://doi.org/10.1029/2020JC016560, 2021. a
Ubelmann, C., Carrere, L., Durand, C., Dibarboure, G., Faugère, Y., Ballarotta, M., Briol, F., and Lyard, F.: Simultaneous estimation of ocean mesoscale and coherent internal tide sea surface height signatures from the global altimetry record, Ocean Sci., 18, 469–481, https://doi.org/10.5194/os-18-469-2022, 2022. a
Veneziani, M., Griffa, A., Reynolds, A. M., and Mariano, A. J.: Oceanic Turbulence and Stochastic Models from Subsurface Lagrangian Data for the Northwest Atlantic Ocean, J. Phys. Oceanogr., 34, 1884–1906, https://doi.org/10.1175/1520-0485(2004)034<1884:OTASMF>2.0.CO;2, 2004. a
Wang, H. and Bühler, O.: Anisotropic Statistics of Lagrangian Structure Functions and Helmholtz Decomposition, J. Phys. Oceanogr., 51, 1375–1393, 2021. a
Wortham, C. and Wunsch, C.: A Multidimensional Spectral Description of Ocean Variability, J. Phys. Oceanogr., 44, 944–966, https://doi.org/10.1175/JPO-D-13-0113.1, 2014. a, b, c, d
Wunsch, C.: Toward a Midlatitude Ocean Frequency–Wavenumber Spectral Density and Trend Determination, J. Phys. Oceanogr., 40, 2264–2281, https://doi.org/10.1175/2010JPO4376.1, 2010. a
Yu, X., Naveira Garabato, A. C., Martin, A. P., Buckingham, C. E., Brannigan, L., and Su, Z.: An Annual Cycle of Submesoscale Vertical Flow and Restratification in the Upper Ocean, J. Phys. Oceanogr., 49, 1439–1461, https://doi.org/10.1175/JPO-D-18-0253.1, 2019a. a
Yu, X., Ponte, A. L., Elipot, S., Menemenlis, D., Zaron, E. D., and Abernathey, R.: Surface Kinetic Energy Distributions in the Global Oceans From a High-Resolution Numerical Model and Surface Drifter Observations, Geophys. Res. Lett., 46, 9757–9766, https://doi.org/10.1029/2019GL083074, 2019b. a, b
Zang, X. and Wunsch, C.: Spectral Description of Low-Frequency Oceanic Variability, J. Phys. Oceanogr., 31, 3073–3095, https://doi.org/10.1175/1520-0485(2001)031<3073:SDOLFO>2.0.CO;2, 2001. a
Zhang, H., Prater, M. D., and Rossby, T.: Isopycnal Lagrangian statistics from the North Atlantic Current RAFOS float observations, J. Geophys. Res.-Oceans, 106, 13817–13836, https://doi.org/10.1029/1999JC000101, 2001. a